Introduction
The release of Llama3, a powerful open-source language model created by Meta, has brought excitement to the world of AI. This model, available in 8B and 70B pretrained and instruction-tuned variants, offers a wide range of applications. In this guide, we will delve into the capabilities of Llama3 and how to access it with Flask, focusing on its potential to revolutionize Generative AI.
Learning Objectives
- Explore the architecture and training methodologies behind Llama3, uncovering its innovative pretraining data and fine-tuning techniques essential for understanding its exceptional performance.
- Experience hands-on implementation of Llama3 through Flask, mastering the art of text generation using transformers while gaining insights into critical aspects of safety testing and tuning.
- Analyze the impressive capabilities of Llama3, including its enhanced accuracy, adaptability, and robust scalability, while also recognizing its limitations and potential risks crucial for responsible use and development.
- Engage with real-world examples and use cases of Llama3, empowering you to leverage its power effectively in diverse applications and scenarios, thereby unlocking its full potential in the realm of Generative AI.
This article was published as a part of the Data Science Blogathon.
Llama3 Architecture and Training
Llama3 is an auto-regressive language model that leverages an optimized transformer architecture. The model was pretrained on an extensive corpus of over 15 trillion tokens of data from publicly available sources, with a cutoff of March 2023 for the 8B model and December 2023 for the 70B model. It employs supervised fine-tuning (SFT) and reinforcement learning with human feedback (RLHF) to align with human preferences for helpfulness and safety.
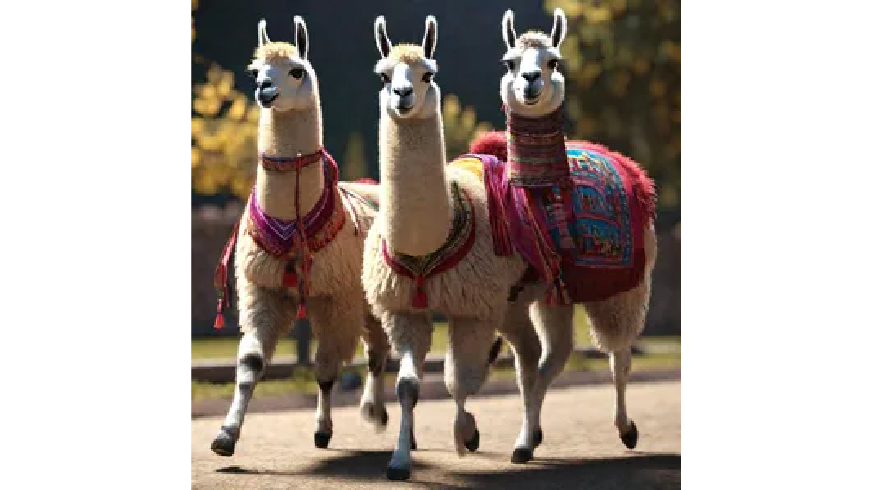
Llama3 Impressive Capabilities
Llama3, with its optimized transformer design, comes in 8B and 70B parameters, in both pre-trained and instruction-tuned versions. It has proven to be remarkably capable in terms of enhanced accuracy, adaptability, robust scalability, and coding capabilities. The model’s open-source and free nature make it accessible to developers without a significant financial investment.
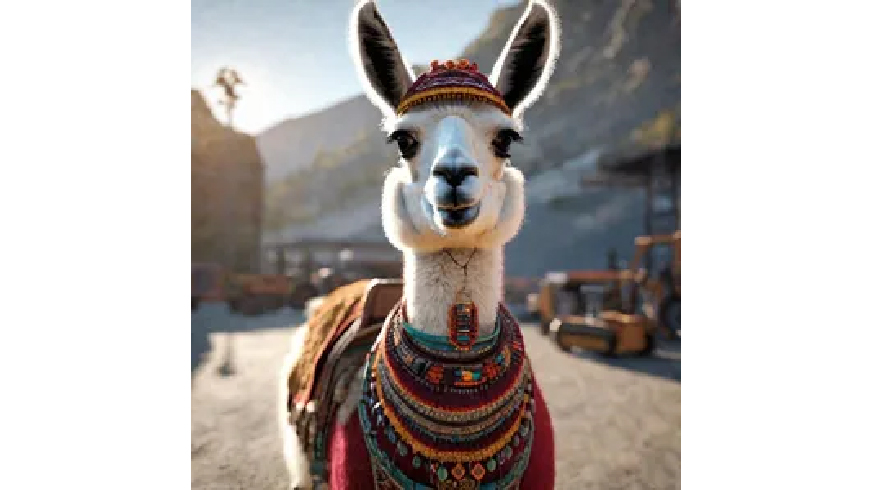
Llama3 Variants and Features
Llama3 offers two major variants, each catering to different use cases with 8B and 70B sizes:
- Pre-trained models: Suitable for natural language generation tasks.
- Instruction-tuned models: Optimized for dialogue use cases.
Llama3 Training Data and Benchmarks
Llama3 was pre-trained on an extensive corpus of over 15 trillion tokens of publicly available data. The fine-tuning data includes publicly available instruction datasets and over 10 million human-annotated examples. The model has achieved impressive results on standard automatic benchmarks.
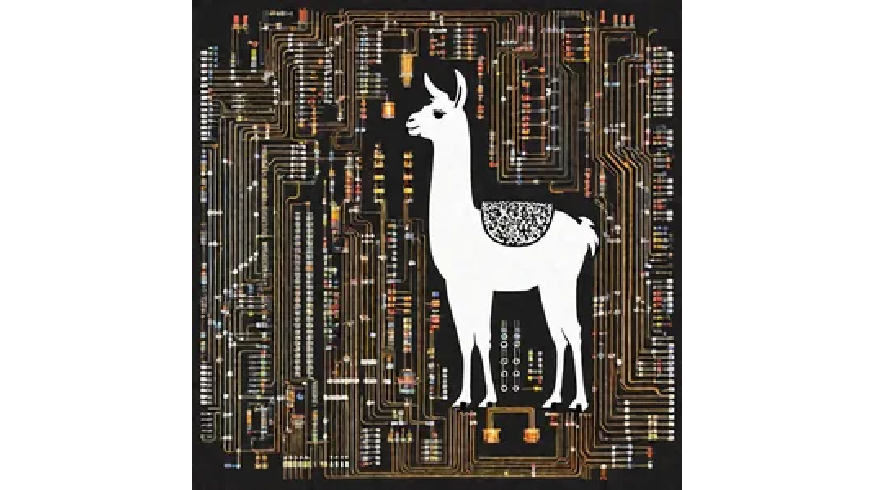
Llama3 Use Cases and Examples
Llama3 can be used with ease like other Llama family models. To access Llama3 with Flask, we will need to follow certain steps. Let’s explore how to access Llama3 with Flask.
How to Access Llama3 with Flask?
Let’s dive into the steps to access Llama3 with Flask:
Step 1: Set up Python Environment
Create a virtual environment (optional but recommended):
$ python -m venv env
$ source env/bin/activate # On Windows use `.\env\Scripts\activate`
Install necessary packages:
We install transformer and accelerate directly from GitHub for the new Llama3 model:
(env) $ pip install -q git+https://github.com/huggingface/transformers.git
(env) $ pip install -q flask transformers torch accelerate # datasets peft bitsandbytes
Step 2: Prepare Main Application File
Create a new Python file called main.py.
Within the document, insert the provided code:
from flask import Flask, request, jsonify
import transformers
import torch
app = Flask(__name__)
# Initialize the model and pipeline outside of the function to avoid unnecessary reloading
model_id = "meta-llama/Meta-Llama-3-8B-Instruct"
pipeline = transformers.pipeline(
"text-generation",
model=model_id,
model_kwargs={"torch_dtype": torch.bfloat16},
device_map="auto",
)
@app.route('/generate', methods=['POST'])
def generate():
data = request.get_json()
user_message = data.get('message')
if not user_message:
return jsonify({'error': 'No message provided.'}), 400
# Create system message
messages = [{"role": "system", "content": "You are a pirate chatbot who always responds in pirate speak!"}]
# Add user message
messages.append({"role": "user", "content": user_message})
prompt = pipeline.tokenizer.apply_chat_template(
messages,
tokenize=False,
add_generation_prompt=True
)
terminators = [
pipeline.tokenizer.eos_token_id,
pipeline.tokenizer.convert_tokens_to_ids("<|eot_id|>")
]
outputs = pipeline(
prompt,
max_new_tokens=256,
eos_token_id=terminators,
do_sample=True,
temperature=0.6,
top_p=0.9,
)
generated_text = outputs[0]['generated_text'][len(prompt):].strip()
response = {
'message': generated_text
}
return jsonify(response), 200
if __name__ == '__main__':
app.run(debug=True)
The code above sets up a Flask web server with a route, /generate, for processing user messages and returning AI-generated responses.
Step 3: Start Flask Application
Launch the Flask app by running the following command:
(env) $ export FLASK_APP=main.py
(env) $ flask run --port=5000
Your Flask app should now be running at http://localhost:5000. You can test the API using tools like Postman or CURL, or create a simple HTML frontend.
Interactive Mode Using Transformers AutoModelForCausalLM
To interactively interact with the model in a Jupyter Notebook, paste the following code into a cell and execute it:
import requests
import sys
sys.path.insert(0,'..')
import torch
from transformers import AutoTokenizer, AutoModelForCausalLM
MODEL_NAME ='meta-llama/Meta-Llama-3-8B-Instruct'
class InteractivePirateChatbot:
def __init__(self):
self._tokenizer = AutoTokenizer.from_pretrained(MODEL_NAME, padding_side="left")
self._tokenizer.pad_token = self._tokenizer.eos_token
self._model = AutoModelForCausalLM.from_pretrained(MODEL_NAME, torch_dtype=torch.bfloat16, device_map="auto", offload_buffers=True)
def _prepare_inputs(self, messages):
try:
inputs = self._tokenizer([message['content'] for message in messages], padding='longest', truncation=True, max_length=512, return_tensors="pt")
input_ids = inputs.input_ids.to(self._model.device)
attention_mask = inputs.attention_mask.to(self._model.device)
return {'input_ids': input_ids, 'attention_mask': attention_mask}
except Exception as e:
print(f"Error preparing inputs: {e}")
return None
def ask(self, question):
try:
messages = [
{"role": "system", "content": "You are a pirate chatbot who always responds in pirate speak!"},
{"role": "user", "content": question}
]
prepared_data = self._prepare_inputs(messages)
if prepared_data is None:
print("Error preparing inputs. Skipping...")
return
output = self._model.generate(**prepared_data, max_length=512, num_beams=5, early_stopping=True)
answer = self._tokenizer.decode(output[0], skip_special_tokens=True)
print("Pirate:", answer)
except Exception as e:
print(f"Error generating response: {e}")
generator = InteractivePirateChatbot()
while True:
question = input("User: ")
generator.ask(question)
This code enables interactive exploration of the model’s capabilities. The full code can be found here.
User: "Who are you?"
Pirate: "Arrrr, me hearty! Me name be Captain Chat, the scurviest pirate chatbot to ever sail the Seven Seas! I be here to swab yer decks with me clever responses and me trusty parrot, Polly, perched on me shoulder. So hoist the colors, me matey, and let's set sail fer a swashbucklin' good time!"
While we have explored the model’s functionality, it’s crucial to address safety and responsibility considerations.
Responsibility and Safety
Meta has implemented various measures to ensure responsible AI development, including safety best practices, Meta Llama Guard 2 and Code Shield safeguards, and updating the Responsible Use Guide. Developers are advised to configure and deploy these safeguards as needed, weighing the benefits of alignment and utility for their specific use case and audience. All relevant links are provided in the Hugging Face repository for Llama3.
Ethical Considerations and Limitations
While Llama3 is a powerful tool, it’s important to recognize its limitations and potential risks. The model may generate inaccurate, biased, or inappropriate responses to user inputs. Therefore, developers should conduct safety testing and tuning tailored to their specific application of the model.
Meta suggests integrating Purple Llama solutions, specifically Llama Guard, into workflows to add an extra layer of system-level safety on top of model-level safety.
Conclusion
Meta has revolutionized artificial intelligence with the introduction of Llama3, a powerful open-source language model. Available in both 8B and 70B pretrained and instruction-tuned versions, Llama3 offers endless possibilities for innovation. This guide delves into Llama3’s capabilities and provides insights on accessing it with Flask, highlighting its potential to redefine Generative AI.
Key Takeaways
– Meta developed Llama3, an open-source language model available in 8B and 70B pretrained and instruction-tuned versions.
– Llama3 showcases enhanced accuracy, adaptability, and scalability.
– The model is open-source and free, making it accessible to developers and researchers.
– Users can utilize Llama3 with transformers for tasks like chatbots and content generation.
– Llama3 and Flask enable exploration of new frontiers in Generative AI, pushing the boundaries of human-machine interaction.
Frequently Asked Questions
Q1. What is Llama3?
A. Meta developed Llama3, a powerful open-source language model available in 8B and 70B pre-trained and instruction-tuned versions.
Q2. What are the key features of Llama3?
A. Llama3 boasts enhanced accuracy, adaptability, and scalability, delivering context-aware responses.
Q3. Is Llama3 open-source and free for commercial use?
A. Llama3 is open-source and free, but review licensing terms for commercial use compliance.
Q4. Can Llama3 be fine-tuned for specific use cases?
A. Yes, adjust hyperparameters and training data to fine-tune Llama3 for specific tasks.
Q5. How does Llama3 compare to other language models like BERT and RoBERTa?
A. Llama3, trained on a larger dataset, surpasses BERT and RoBERTa in various natural language processing tasks.
Links
The media in this article is used at the author’s discretion.
[Author: Mobarak Inuwa]