Introduction
Agriculture has always been a cornerstone of human civilization, providing sustenance and livelihoods for billions worldwide. As technology advances, we find new and innovative ways to enhance agricultural practices. One such advancement is using Vision Transformers (ViTs) to classify leaf diseases in crops. In this blog, we’ll explore how vision transformers in agriculture revolutionize by offering an efficient and accurate solution for identifying and mitigating crop diseases.
Cassava, or manioc or yuca, is a versatile crop with various uses, from providing dietary staples to industrial applications. Its hardiness and resilience make it an essential crop for regions with challenging growing conditions. However, cassava plants are vulnerable to various diseases, with CMD and CBSD being among the most destructive.
CMD is caused by a complex of viruses transmitted by whiteflies, leading to severe mosaic symptoms on cassava leaves. CBSD, on the other hand, is caused by two related viruses and primarily affects storage roots, rendering them inedible. Identifying these diseases early is crucial for preventing widespread crop damage and ensuring food security. Vision Transformers, an evolution of the transformer architecture initially designed for natural language processing (NLP), have proven highly effective in processing visual data. These models process images as sequences of patches, using self-attention mechanisms to capture intricate patterns and relationships in the data. In the context of cassava leaf disease classification, ViTs are trained to identify CMD and CBSD by analyzing images of infected cassava leaves.
Learning Outcomes
- Understanding Vision Transformers and how they are applied to agriculture, specifically for leaf disease classification.
- Learn about the fundamental concepts of the transformer architecture, including self-attention mechanisms, and how these are adapted for visual data processing.
- Understand the innovative use of Vision Transformers (ViTs) in agriculture, specifically for the early detection of cassava leaf diseases.
- Gain insights into the advantages of Vision Transformers, such as scalability and global context, as well as their challenges, including computational requirements and data efficiency.
This article was published as a part of the Data Science Blogathon.
The Rise of Vision Transformers
Computer vision has made tremendous strides in recent years, thanks to the development of convolutional neural networks (CNNs). CNNs have been the go-to architecture for various image-related tasks, from image classification to object detection. However, Vision Transformers have risen as a strong alternative, offering a novel approach to processing visual information. Researchers at Google Research introduced Vision Transformers in 2020 in a groundbreaking paper titled “An Image is Worth 16×16 Words: Transformers for Image Recognition at Scale.” They adapted the transformer architecture, initially designed for natural language processing (NLP), to the domain of computer vision. This adaptation has opened up new possibilities and challenges in the field.
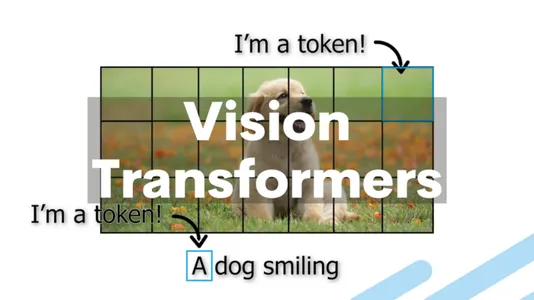
The use of ViTs offers several advantages over traditional methods, including:
- High Accuracy: ViTs excel in accuracy, allowing for the reliable detection and differentiation of leaf diseases.
- Efficiency: Once trained, ViTs can process images quickly, making them suitable for real-time disease detection in the field.
- Scalability: ViTs can handle datasets of varying sizes, making them adaptable to different agricultural settings.
- Generalization: ViTs can generalize to different cassava varieties and disease types, reducing the need for specific models for each scenario.
The Transformer Architecture: A Brief Overview
Before diving into Vision Transformers, it’s essential to understand the core concepts of the transformer architecture. Transformers, originally designed for NLP, revolutionized language processing tasks. The key features of transformers are self-attention mechanisms and parallelization, allowing for more comprehensive context understanding and faster training.
At the heart of transformers is the self-attention mechanism, which enables the model to weigh the importance of different input elements when making predictions. This mechanism, combined with multi-head attention layers, captures complex relationships in data.
So, how do Vision Transformers apply this transformer architecture to the domain of computer vision? The fundamental idea behind Vision Transformers is to treat an image as a sequence of patches, just as NLP tasks treat text as a sequence of words. The transformer layers then process each patch in the image by embedding it into a vector.
Key Components of a Vision Transformer
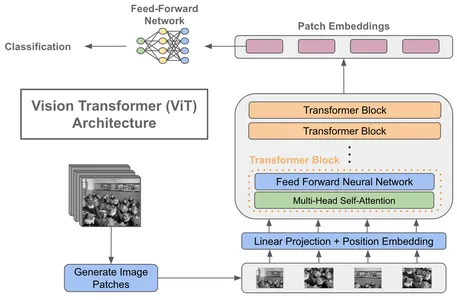
- Patch Embeddings: Divide an image into fixed-size, non-overlapping patches, typically 16×16 pixels. Each patch is then linearly embedded into a lower-dimensional vector.
- Positional Encodings: Add Positional encodings to the patch embeddings to account for the spatial arrangement of patches. This allows the model to learn the relative positions of patches within the image.
- Transformer Encoder: Vision Transformers consist of multiple transformer encoder layers like NLP transformers. Each layer performs self-attention and feed-forward operations on the patch embeddings.
- Classification Head: At the end of the transformer layers, a classification head is added for tasks like image classification. It takes the output embeddings and produces class probabilities.
The introduction of Vision Transformers marks a significant departure from CNNs, which rely on convolutional layers for feature extraction. By treating images as sequences of patches, Vision Transformers achieve state-of-the-art results in various computer vision tasks, including image classification, object detection, and even video analysis.
Implementation
Dataset
The Cassava Leaf Disease dataset comprises around 15,000 high-resolution images of cassava leaves exhibiting various stages and degrees of disease symptoms. Each image is meticulously labeled to indicate the disease present, allowing for supervised machine learning and image classification tasks. Cassava diseases exhibit distinct characteristics, leading to their classification into several categories. These categories include Cassava Bacterial Blight (CBB), Cassava Brown Streak Disease (CBSD), Cassava Green Mottle (CGM), and Cassava Mosaic Disease (CMD). Please rephrase this sentence. Please rewrite the following sentence. Can you please rephrase this?