As the global energy demand continues to rise, companies in the oil and gas sector are under increasing pressure to improve operational efficiency, reduce costs, and comply with safety and environmental regulations. Artificial intelligence (AI) has emerged as a key solution to meet these challenges. The integration of AI into the oil and gas industry over the past decade has brought about significant transformations.
This article will delve into the market dynamics of AI in the oil and gas industry, focusing on major trends, driving factors, challenges, and opportunities.
The technological state of the oil and gas industry in 2024
In 2024, the oil and gas industry is being shaped by the adoption of AI and the increasing emphasis on sustainability.
A report titled “Transforming the Energy Industry with AI” by MIT Technology Review and Siemens Energy showcases several case studies demonstrating how AI is rapidly changing the oil and gas sector.
For instance, Abu Dhabi National Oil Company (ADNOC) has utilized AI to enhance planning, scheduling, and safety measures, resulting in a 20% reduction in maintenance costs. Prior to implementing digital solutions, ADNOC’s production planning process was laborious and time-consuming, involving multiple Excel spreadsheets. By creating digital twins of assets and plants, ADNOC can now conduct rapid scenario analysis and make informed decisions. This digital transformation has enabled ADNOC to extend maintenance cycles for key equipment such as turbines and pumps, with these updates reflected in their maintenance policies.
Chevron, on the other hand, is focusing on AI integration across various operations, from petrotechnical applications to cybersecurity. The company is also committed to environmental conservation, employing advanced deep learning techniques in computer vision and bioacoustics for wildlife protection initiatives.
The application of AI in the oil and gas industry is projected to expand in the coming years. A recent survey by EY revealed that over 92% of global oil and gas companies are either currently investing in AI or planning to do so in the near future. Here are some key statistics from the research agency Future Market Insights:
- The AI market in the oil and gas sector is expected to grow at a rate (CAGR) of 14.1%, reaching a value of US$ 13 billion by 2034.
- The solution segment is predicted to lead the global market, with a projected CAGR of 13.8% from 2024 to 2034.
- The upstream segment is anticipated to drive the global market in 2024, with a projected CAGR of 13.6%.
- Experts forecast that the AI market for the oil and gas industry in the United States will grow at an annual rate of 14.5% up to 2034.
The primary drivers behind this growth are linked to the need for improved operational efficiency and the necessity to compete with technologically advanced rivals.
- Operational efficiency and cost reduction: AI technologies like machine learning and data analytics help optimize production and identify cost-saving opportunities.
- Enhanced safety and environmental sustainability: AI aids in risk identification and mitigation, aiming to reduce accidents and minimize emissions and waste.
- Data utilization: The availability of extensive sensor data enhances decision-making and operational enhancements through AI analysis and forecasting.
- Competitive investment: Established corporations and startups alike are heavily investing in AI to develop innovative solutions tailored for the oil and gas industry, intensifying competition in the market.
Key trends of AI adoption in oil and gas
The major areas where AI is increasingly being applied by industry leaders in the oil and gas sector include:
- Predictive maintenance: The utilization of AI algorithms for predictive maintenance is on the rise, aimed at preventing equipment failures and minimizing downtime.
- Digital twins: This technology creates virtual models of physical assets, enabling real-time monitoring and simulation of operations.
- Edge computing: There is a growing shift towards edge computing, which processes data directly at its source (such as offshore platforms) to reduce latency and enhance real-time decision-making.
- Partnerships with technology leaders: Large oil and gas enterprises are forming partnerships with tech giants and AI startups to leverage advanced AI technologies and specialized expertise.
We will delve deeper into each trend in the following sections.
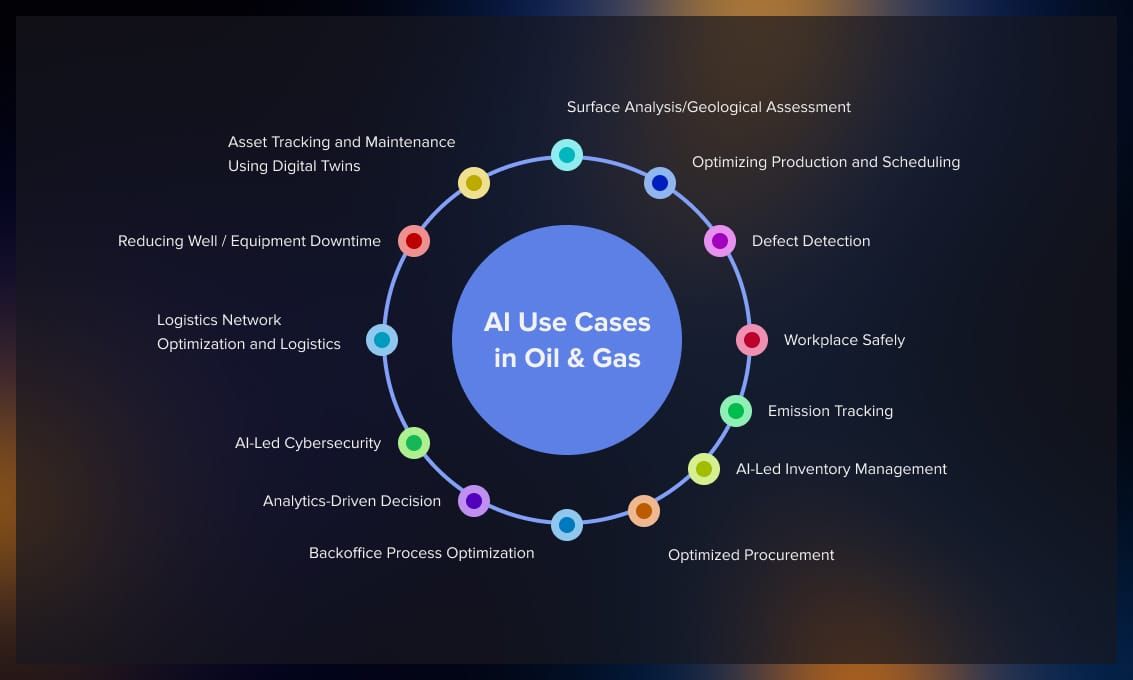
Trend 1. Predictive maintenance
AI-driven predictive maintenance involves using artificial intelligence technologies to predict equipment failures, optimize maintenance schedules, and reduce operational downtime. This approach contrasts with traditional reactive maintenance, which responds to equipment failures after they occur, or preventive maintenance, which follows a predetermined schedule regardless of actual equipment condition.
Core components
- Data collection: Sensors installed on equipment gather real-time data on operational parameters such as temperature, pressure, vibration, and flow rates.
- Data integration: This data is combined with historical maintenance records, operational data, and environmental condition indicators to create a comprehensive dataset.
- Machine learning models: ML models analyze the data to identify patterns, anomalies, and trends.
- Predictive analytics: The system predicts potential equipment failures and recommends optimal maintenance schedules based on the analysis.
Trend 2. Digital twins in the oil and gas industry
Digital twins in the oil and gas sector are virtual representations of physical assets, processes, or systems used in exploration, extraction, processing, and distribution. They enable informed decision-making, operational optimization, and innovation while mitigating risks.
This technology leverages data analytics, machine learning, and simulation to create a dynamic digital replica of physical entities. These replicas can operate in parallel with their real-world counterparts, providing real-time monitoring, analysis, and prediction.
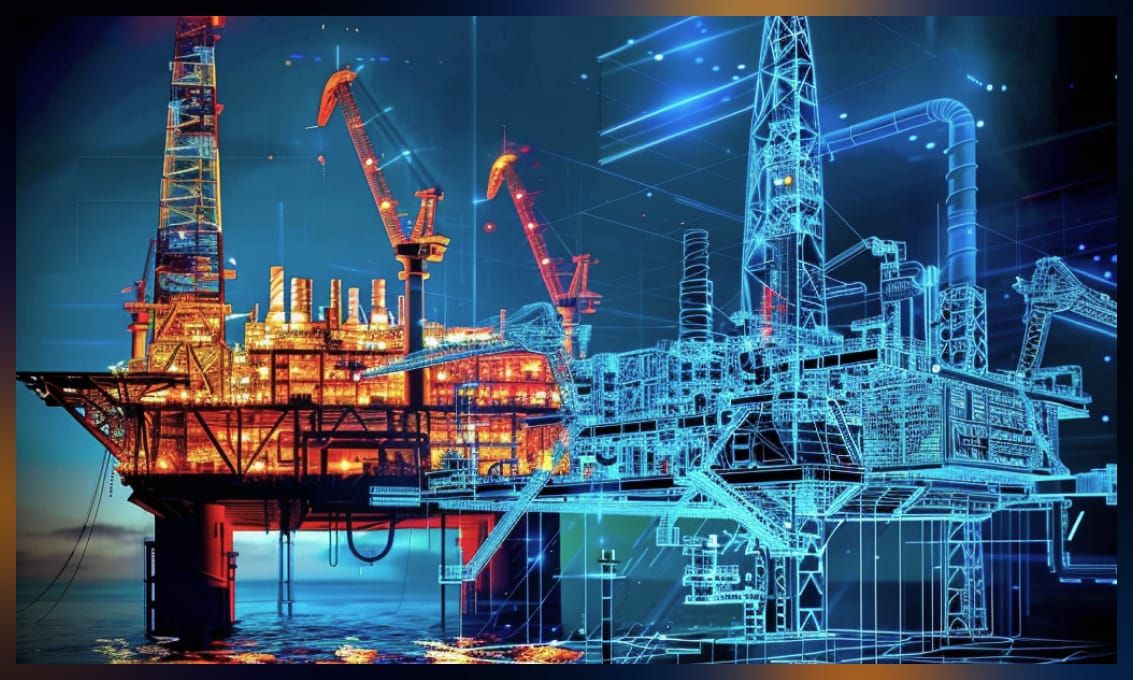
Design and development
In the design and development phase, digital twins can simulate the behavior of infrastructure or equipment under various conditions. Making necessary adjustments before physical assets are built or deployed allows for proactive risk reduction, control of equipment vulnerabilities, and resource optimization.
Operational efficiency
By simulating oil and gas processes, digital twins can help identify bottlenecks, optimize workflows, and reduce operational costs. This includes everything from drilling and extraction to transportation and refining processes.
Risk management
Digital twins play a significant role in risk management by predicting the outcomes of potential hazardous events such as equipment failures, leaks, or environmental impacts. This enables companies to proactively mitigate risks and ensure safety and compliance with regulations.
Innovation and experimentation
Digital twins allow companies to experiment with new exploration, extraction, and processing methods without the risks and costs of physical testing by simulating and testing scenarios in a virtual environment.
Training and development
Digital twins provide a realistic and interactive platform for training employees on complex oil and gas operations without the need for physical presence, enhancing safety and efficiency.
Trends 3. Edge computing in oil and gas
Edge computing involves deploying data processing equipment closer to the data source, such as remote drilling sites, offshore platforms, or pipelines. This differs from cloud computing, where data is processed at distant data centers.
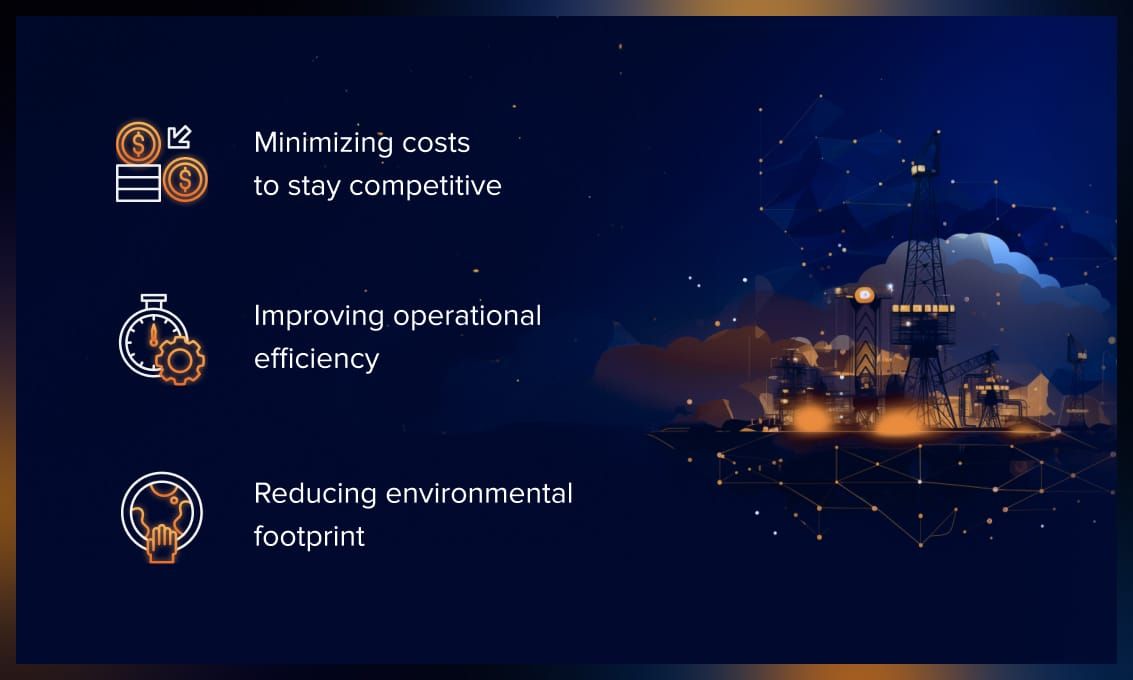
Real-time data processing
In remote or offshore operations, connectivity may be limited or unreliable. Edge computing allows for processing large amounts of data generated by sensors and equipment directly at the source. This enables real-time monitoring and analysis of critical operational parameters like pressure, temperature, and flow rates.
Safety and environmental monitoring
Edge computing enables real-time monitoring of environmental conditions and the detection of hazardous situations such as gas leaks or structural failures. Quick local data processing ensures immediate alerts and responses, significantly enhancing safety protocols and environmental protection measures.
Operational optimization
By processing data locally, companies can optimize operations in real time, adjusting drilling parameters, production rates, and resource allocation based on immediate feedback from the edge. This leads to more efficient resource utilization, optimized production, and reduced operational costs.
Bandwidth and connectivity savings
Transmitting large volumes of data from remote sites to centralized data centers requires significant bandwidth and can incur high costs. Edge computing reduces the need for data transmission, lowering bandwidth requirements and connectivity costs.
Enhanced security
Edge computing enhances data security by processing sensitive information locally.
Implementation considerations
Implementing edge computing in the oil and gas industry requires addressing challenges such as ensuring the reliability and robustness of edge computing devices in harsh environments, managing the deployment and maintenance of these systems, and integrating edge computing into existing IT and operational technology infrastructures.
Trend 4. Strategic partnerships between oil and gas and tech companies
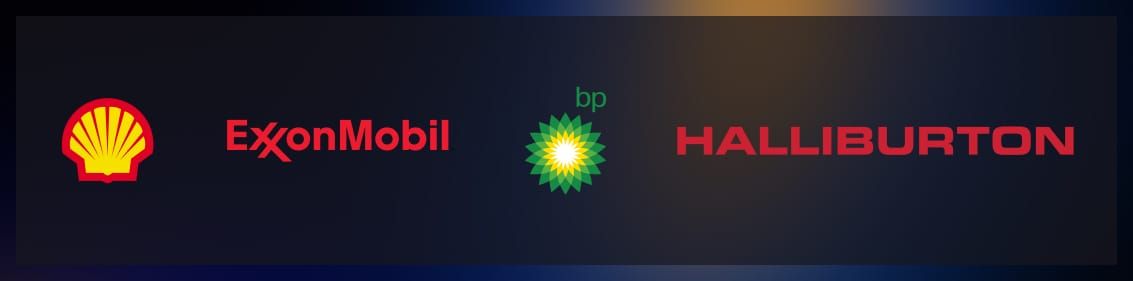
Major oil and gas companies are increasingly collaborating with technology leaders, including tech giants and AI startups, to enhance exploration, safety, production efficiency, and sustainability in the oil and gas sector.
Shell
Shell has partnered with Microsoft to accelerate its digital transformation and innovation in energy efficiency and carbon emissions reduction. This strategic alliance involves leveraging AI and other digital tools to support Shell’s business operations, from exploration and production to energy and product distribution. Microsoft’s cloud services, AI capabilities, and IoT solutions play a crucial role in optimizing the oil company’s operations and enabling more sustainable energy solutions.
Shell is currently working on 160 active AI-driven projects across its oil and gas supply chain. These projects include exploration and drilling initiatives that utilize reinforcement learning techniques to minimize gas extraction costs. Additionally, Shell’s automated drilling systems, trained using proprietary and simulated data, assist drill operators in gaining a deeper understanding of the underground environment.
In response to the growing electric vehicle (EV) market, Shell’s RechargePlus program utilizes AI to forecast fluctuating demand for EV charging stations, ensuring efficient energy supply. Moreover, in Singapore and Thailand, Shell employs computer vision technology at its retail stations to detect unsafe behaviors, such as smoking near pumps, allowing staff to quickly shut down pumps to prevent accidents.
BP
BP has forged a significant partnership with Microsoft since 2017, adopting Azure cloud solutions and machine learning to enhance drilling efficiency. This collaboration has enabled BP to centralize its data centers on Azure, streamlining data analysis and decision-making, resulting in a substantial reduction in drilling times. In 2020, BP also partnered with Bluware in the field of deep learning, aiming to simplify the interpretation of subsurface data, a process traditionally marked by manual labor and extensive time investment.
BP’s investment in AI extends to several companies, including Grid Edge and Belmont Technologies. Grid Edge offers an AI-powered energy management platform that optimizes energy profiles for buildings, while Belmont Technologies provides industrial AI software for critical operational insights, process automation, and optimization. Other notable investments include Beyond Limits and Satelytics, with AI applications ranging from locating oil and gas reserves to environmental monitoring and risk management.
BP has also explored other uses of AI, such as deploying the Spot robot in refineries to collect data, detect abnormalities, and monitor emissions, reducing human exposure to hazardous conditions.
ExxonMobil
ExxonMobil is another industry leader leveraging AI. The company collaborates with IBM’s Data Science and AI Elite Team and seismic experts to enhance data integration and interpretation. This has resulted in a unified data repository hosted on a multi-cloud environment. ExxonMobil’s partnership with Fiserv introduces voice-activated payments at gas stations using Alexa and Echo Auto, processed via Amazon Pay. Additionally, in collaboration with MIT’s AI lab, ExxonMobil is developing AI-driven, self-learning submersible robots for ocean exploration.
Halliburton
Halliburton is a leader in the application of artificial intelligence for oil exploration. The company’s AI-based DS365.ai software enhances various aspects of oil exploration, from subsurface analysis to drilling and production. In 2022, Halliburton teamed up with the Saudi Data and Artificial Intelligence Authority (SDAIA) to design advanced solutions using the DS365.ai software. Moreover, Halliburton’s LOGIX Autonomous Drilling Platform leverages AI and ML to optimize the performance of mature brownfield oil wells.
What are the challenges of AI implementation?
Despite the significant potential of AI in the oil and gas industry, its implementation faces several challenges:
- Data quality: Ensuring data quality and consistency is crucial for AI applications. Flaws in data can lead to inaccurate insights and wrong decisions.
- Legacy systems compatibility: Many oil and gas companies operate on legacy systems that may not seamlessly integrate with advanced AI technologies. Upgrading or replacing these systems while maintaining operational continuity can be a significant challenge.
- Initial investment costs: Adopting AI technology requires large-scale investments in technology, infrastructure, and a skilled workforce.
- Talent shortage: There is a notable shortage of professionals with AI expertise specific to the oil and gas industry, making it challenging to secure and maintain a qualified workforce.
- Regulatory compliance: The oil and gas industry is heavily regulated, and AI implementation must comply with strict industry standards and government regulations. Ensuring that AI solutions adhere to these regulations can be a complex process.
Sustainability
Many oil and gas companies are actively working to reduce their carbon footprint and make their operations more environmentally friendly, with AI playing a crucial role in these efforts. One example is the partnership between Occidental Petroleum, a US oil and gas company, and Carbon Engineering, a Canadian startup, to establish a facility designed to capture and store half a million metric tons of CO₂ annually.
This shift towards sustainability is not only driven by environmental concerns but also by the increasing trend among investors to support environmental initiatives in the industry. For example, Climate Action 100+, an initiative launched in 2017 to address climate issues, has over 700 participants managing assets exceeding $68 trillion. Governments are also prioritizing sustainable investments as key components of their economic recovery plans.
Additionally, research by the Boston Consulting Group highlights the substantial potential of AI in enhancing corporate sustainability. By 2030, the use of AI could generate between $1.3 and $2.6 trillion in value through new revenue streams and cost savings.
The statistics mentioned above indicate that companies that do not adopt new approaches today may risk falling behind in the future. To enhance their financial stability and reputation, and capitalize on the transition towards a low-carbon future, companies must transform their operations through the integration of AI.
Conclusion
In conclusion, the integration of artificial intelligence into the oil and gas industry represents a significant shift towards addressing the complex challenges of today’s energy landscape. AI not only provides tools to enhance operational efficiency, extend equipment lifespan, and reduce costs but also plays a critical role in ensuring safety and environmental compliance. As the industry continues to evolve, embracing AI technologies will be essential for companies looking to stay competitive in an increasingly demanding and dynamic global market.