Introduction
Retrieval Augmented Generation systems, also known as RAG systems, have gained popularity for creating Generative AI assistants using custom enterprise data. They offer a cost-effective alternative to fine-tuning Large Language Models (LLMs). One of the main benefits of RAG systems is their ability to integrate data seamlessly, enhance the intelligence of LLMs, and provide more contextual answers to queries. However, there are challenges that can cause RAG systems to underperform and even provide incorrect responses. In this guide, we explore how AI Agents can enhance the capabilities of traditional RAG systems and address some of their limitations.
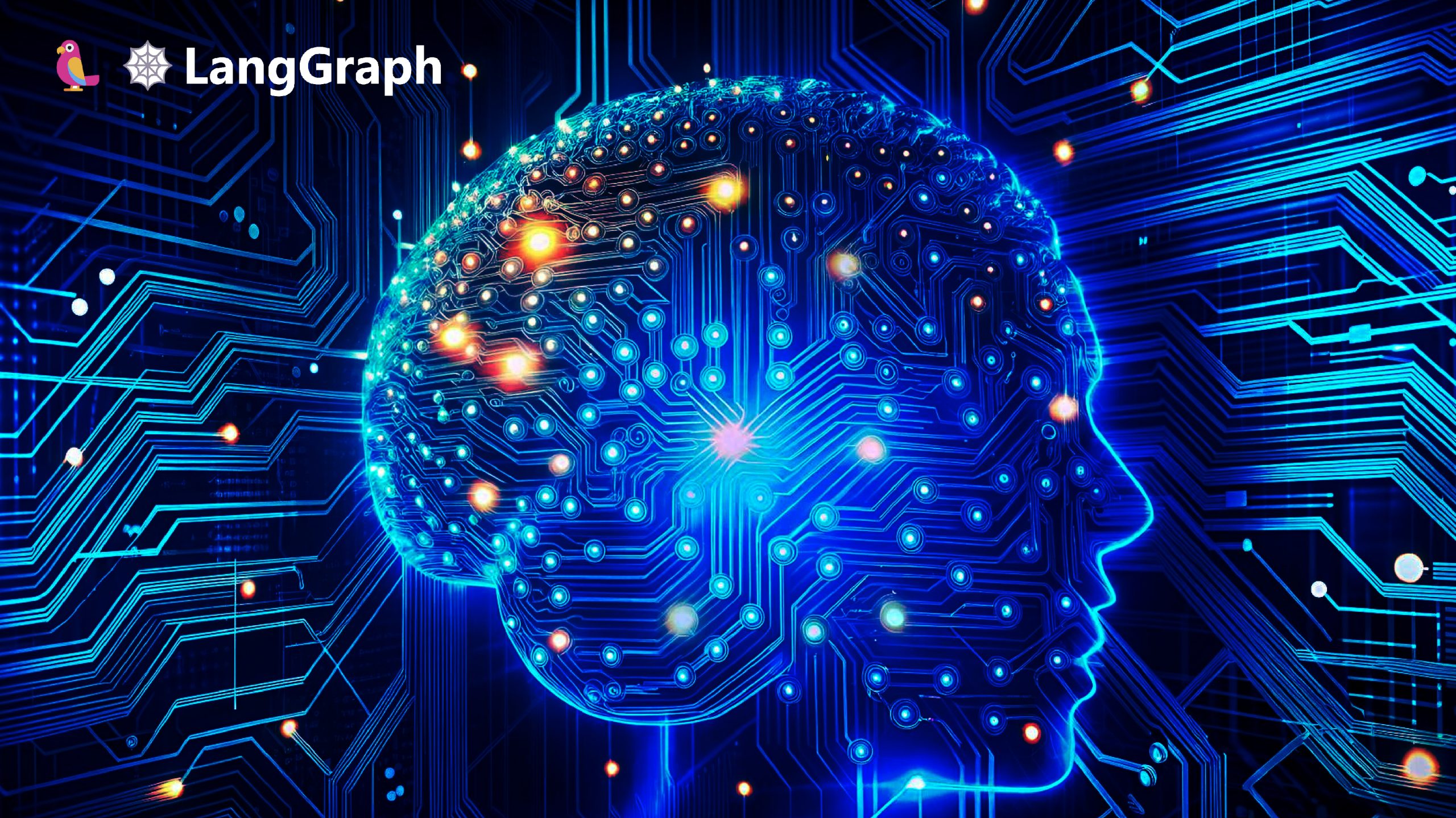
Overview
- Traditional RAG systems face challenges such as lack of real-time data and potential retrieval of irrelevant documents.
- The proposed Agentic Corrective RAG system utilizes AI agents to enhance RAG capabilities and overcome limitations.
- It includes a document grading step to assess the relevance of retrieved documents to the query.
- If irrelevant documents are retrieved, the system rephrases the query and conducts a web search for current information.
- The system utilizes LangGraph to incorporate components like document retrieval, grading, query rewriting, and web search.
- Its objective is to provide more accurate and timely responses by combining static knowledge with real-time web data.
- The implementation demonstrates improved performance for queries requiring current information or beyond the initial knowledge base.
Traditional RAG System Architecture
A typical Retrieval Augmented Generation (RAG) system architecture consists of two main steps:
- Data Processing and Indexing
- Retrieval and Response Generation
Step 1: Data Processing and Indexing
In the first step of Data Processing and Indexing, the focus is on transforming custom enterprise data into a more manageable format by loading text content, tables, and images, segmenting large documents, converting them into embeddings using an embedder model, and storing them in a vector database.
Step 2: Retrieval and Response Generation
In the second step, when a user poses a question, relevant document segments are retrieved from the vector database, sent along with the question to a Large Language Model (LLM) for generating a human-like response.
This two-step workflow is commonly used for traditional RAG system development, but it comes with limitations.
Traditional RAG System Limitations
Some limitations of traditional RAG systems include:
- Lack of access to real-time data
- The system’s efficacy depends on the quality of data in the vector database
- Poor retrieval strategies can result in irrelevant documents used for responses
- LLMs may struggle with generating accurate responses or experience hallucinations
This article focuses on the limitations of RAG systems without real-time data access, and the importance of ensuring retrieved document segments are relevant for providing accurate responses, especially for recent events and real-time data to minimize errors.
Corrective RAG System
Our agentic RAG system is inspired by the solution proposed in the paper, Corrective Retrieval Augmented Generation, Yan et al., which introduces a workflow to enhance a standard RAG system. The approach involves retrieving document segments from the vector database, using an LLM to verify their relevance to the input question.
If all retrieved document segments are relevant, the process continues to response generation by the LLM. However, if some segments are irrelevant, the system rephrases the query, searches the web for new information related to the question, and then generates a response using the LLM.
The key innovation in this method is the inclusion of web searches to enhance the static information in the vector database with real-time data, ensuring the relevance of retrieved documents to the input question beyond simple cosine similarity comparisons.
The Rise of AI Agents
AI Agents or Agentic AI systems have gained prominence, particularly in 2024, enabling the development of Generative AI systems capable of reasoning, analyzing, interacting, and autonomously taking actions. Agentic AI aims to create fully autonomous systems that can understand and manage complex tasks with minimal human intervention. These systems can comprehend intricate concepts, set and pursue goals, reason through tasks, and adapt based on changing conditions. They can be single agents or multiple agents, as depicted below, working together to convert user instructions into functional code snippets.
Various frameworks like CrewAI, LangChain, LangGraph, AutoGen, and others are used to build Agentic AI systems, simplifying the development of complex workflows. An agent comprises one or more LLMs with access to tools to answer user queries based on specific prompts.
For our practical implementation of the Agentic RAG system, we will utilize LangGraph. LangGraph, built on LangChain, allows the creation of cyclic graphs essential for AI agents powered by LLMs, with an interface inspired by the NetworkX library. It enables the coordination and checkpointing of multiple chains through cyclic computational steps.
We enter our Tavily Search API key using the getpass() function to ensure that it is not exposed in the code.
To obtain a free API key, please visit this link.
TAVILY_API_KEY = getpass('Enter Tavily Search API Key: ')
Setting Up Environment Variables
Next, we set up system environment variables for authentication and searching APIs.
import os
os.environ['OPENAI_API_KEY'] = OPENAI_KEY
os.environ['TAVILY_API_KEY'] = TAVILY_API_KEY
Constructing a Vector Database for Wikipedia Data
We will create a vector database using a subset of documents extracted from Wikipedia.
Utilizing Open AI Embedding Models
We can access Open AI embedding models like the text-embedding-3-small and text-embedding-3-large models for converting document chunks into embeddings.
from langchain_openai import OpenAIEmbeddings
openai_embed_model = OpenAIEmbeddings(model="text-embedding-3-small")
Accessing Wikipedia Data
We have the Wikipedia documents available in an archive file; you can download it manually or using the provided code snippet.
If unable to download using the following code, visit the given Google Drive link to download manually and upload it on Google Colab:
Google Drive Link: https://drive.google.com/file/d/1oWBnoxBZ1Mpeond8XDUSO6J9oAjcRDyW
For Google Colab: !gdown 1oWBnoxBZ1Mpeond8XDUSO6J9oAjcRDyW
Loading and Chunking Documents
We will unzip the data archive, load the documents, split them into manageable chunks, and index them.
import gzip
import json
from langchain.docstore.document import Document
from langchain.text_splitter import RecursiveCharacterTextSplitter
# Code snippet for loading and chunking documents
OUTPUT
Sample output of chunked documents
Creating a Vector Database and Saving it
Initialize a connection to a Chroma vector DB client and save the data to disk, using the Open AI embedding model to transform chunks into embeddings.
from langchain_chroma import Chroma
# Code snippet for creating a vector database and saving it
Setting Up a Vector Database Retriever
Utilize the Similarity with Threshold Retrieval strategy to retrieve similar documents based on user queries.
similarity_threshold_retriever = chroma_db.as_retriever(search_type="similarity_score_threshold",
search_kwargs={"k": 3, "score_threshold": 0.3})
# Code snippet for testing the retriever
OUTPUT
Sample output of top 3 similar documents
Creating a Query Retrieval Grader
Use an LLM, GPT-4o, to grade the relevance of retrieved documents to user questions.
# Code snippet for creating a query retrieval grader
Test the grader on sample user queries to assess the relevance of retrieved documents.
# Code snippet for testing the grader
OUTPUT
Sample output of graded documents
Building a QA RAG Chain
Connect the retriever to an LLM, GPT-4o, to construct a Question-Answering RAG chain.
This will be our traditional RAG system, to be integrated with an AI Agent later.
from langchain_core.prompts import ChatPromptTemplate
from langchain_openai import ChatOpenAI
from langchain_core.runnables import RunnablePassthrough, RunnableLambda
from langchain_core.output_parsers import StrOutputParser
from operator import itemgetter
prompt = """You are an assistant for question-answering tasks.
Use the following pieces of retrieved context to answer the question.
If no context is present or if you don't know the answer, just say that you don't know the answer.
Do not make up the answer unless it is there in the provided context.
Give a detailed answer and to the point answer with regard to the question.
Question:
{question}
Context:
{context}
Answer:
"""
prompt_template = ChatPromptTemplate.from_template(prompt)
chatgpt = ChatOpenAI(model_name="gpt-4o", temperature=0)
def format_docs(docs):
return "\n\n".join(doc.page_content for doc in docs)
qa_rag_chain = (
{
"context": (itemgetter('context')
|
RunnableLambda(format_docs)),
"question": itemgetter('question')
}
|
prompt_template
|
chatgpt
|
StrOutputParser()
)
The concept is to receive the user query, retrieve context documents, and input them into the RAG prompt for generating responses using GPT-4o. Let’s test the system with some queries.
query = "what is the capital of India?"
top3_docs = similarity_threshold_retriever.invoke(query)
result = qa_rag_chain.invoke(
{"context": top3_docs, "question": query}
)
print(result)
OUTPUT
The capital of India is New Delhi. It is also a union territory and part of the megacity of Delhi.
Now, let’s try a question without relevant context documents.
query = "who won the champions league in 2024?"
top3_docs = similarity_threshold_retriever.invoke(query)
result = qa_rag_chain.invoke(
{"context": top3_docs, "question": query}
)
print(result)
OUTPUT
I don't know the answer. The provided context does not contain information about the winner of the Champions League in 2024.
The RAG system works as expected but struggles with out-of-context questions. This will be addressed in the next steps.
Also read: Build an AI Coding Agent with LangGraph by LangChain
Create a Query Rephraser
We will develop a query rephraser using an LLM (GPT-4o) to optimize user queries for web searches, enhancing context retrieval.
llm = ChatOpenAI(model="gpt-4o", temperature=0)
SYS_PROMPT = """Act as a question re-writer and perform the following task:
- Convert the following input question to a better version that is optimized for web search.
- When re-writing, look at the input question and try to reason about the underlying semantic intent / meaning.
"""
re_write_prompt = ChatPromptTemplate.from_messages(
[
("system", SYS_PROMPT),
("human", """Here is the initial question:
{question}
Formulate an improved question.
""",
),
]
)
question_rewriter = (re_write_prompt
|
llm
|
StrOutputParser())
Let’s test the rephraser chain with a sample question.
query = "who won the champions league in 2024?"
question_rewriter.invoke({"question": query})
OUTPUT
Who was the winner of the 2024 UEFA Champions League?
We’ll use the Tavily API for web searches and load a connection for this purpose. The top 3 search results will be used for additional context information.
from langchain_community.tools.tavily_search import TavilySearchResults
tv_search = TavilySearchResults(max_results=3, search_depth="advanced", max_tokens=10000)
Build Agentic RAG components
We’ll create essential components for our Agentic Corrective RAG System following the workflow discussed earlier. These functions will be integrated into agent nodes using LangGraph.
Graph State
Used to store and represent the agent graph’s state, tracking user queries, web search necessity, context documents, and LLM responses.
from typing import List
from typing_extensions import TypedDict
class GraphState(TypedDict):
question: str
generation: str
web_search_needed: str
documents: List[str]
Retrieve function for retrieval from Vector DB
Retrieves context documents from the vector database based on the user query stored in the graph state.
def retrieve(state):
question = state["question"]
documents = similarity_threshold_retriever.invoke(question)
return {"documents": documents, "question": question}
Grade documents
Determines the relevance of retrieved documents to the question using an LLM Grader and sets the web_search_needed flag accordingly.
The state graph is updated by ensuring that context documents consist only of relevant documents. The process involves grading the documents using an LLM Grader and filtering out irrelevant ones. If all documents are relevant, no web search is needed. If any document is deemed irrelevant or if the documents are empty, a web search is required to gather more context.
To facilitate this process, several functions are implemented, such as rewriting the query to produce a better question for web search, conducting the actual web search, and generating an answer using the retrieved documents. Additionally, a function is included to decide whether a web search is needed based on the relevance of the existing documents.
These functions are organized into an agentic RAG system using LangGraph, with nodes representing each function and edges connecting them in a logical workflow. The system can be tested with user queries to demonstrate its functionality. We can now proceed to check the response:
“`python
display(Markdown(response[‘generation’]))
“`
**OUTPUT**
“`
The winner of the 2024 UEFA Champions League was Real Madrid. They secured victory in the final against Borussia Dortmund with goals from Dani Carvajal and Vinicius Junior.
“`
Let’s test our last scenario to ensure that the flow works correctly. In this scenario, all retrieved documents from the vector database are relevant to the user query, so ideally, no web search should be conducted.
“`python
query = “Tell me about India”
response = agentic_rag.invoke({“question”: query})
“`
**OUTPUT**
“`
—RETRIEVAL FROM VECTOR DB—
—CHECK DOCUMENT RELEVANCE TO QUESTION—
—GRADE: DOCUMENT RELEVANT—
—GRADE: DOCUMENT RELEVANT—
—GRADE: DOCUMENT RELEVANT—
—ASSESS GRADED DOCUMENTS—
—DECISION: GENERATE RESPONSE—
—GENERATE ANSWER—
“`
Our agentic RAG system is functioning well, as demonstrated in this case where it does not perform a web search since all retrieved documents are relevant for answering the user’s question. Let’s review the response now:
“`python
display(Markdown(response[‘generation’]))
“`
**OUTPUT**
“`
India is a country located in Asia, specifically at the center of South Asia. It is the seventh largest country in the world by area and the largest in South Asia. . . . . . .
India has a rich and diverse history that spans thousands of years, encompassing various languages, cultures, periods, and dynasties. The civilization began in the Indus Valley, . . . . . .
“`
### Conclusion
In this guide, we delved into a comprehensive understanding of the challenges faced by traditional RAG systems, the significance of AI Agents, and how Agentic RAG systems can address some of these challenges. We explored a detailed system architecture and workflow for an Agentic Corrective RAG system inspired by the Corrective Retrieval Augmented Generation paper. Finally, we implemented this Agentic RAG system with LangGraph and tested it in various scenarios. For access to the code and to enhance the system with additional capabilities, such as hallucination checks, refer to [this Colab notebook](https://colab.research.google.com/drive/1MROAG-dl4sBEMueSZ7LiliStSulNhEPA?usp=sharing).
Unlock your potential with the GenAI Pinnacle Program, where you can learn to build Agentic AI systems in detail. Benefit from 1:1 mentorship with Generative AI experts, an advanced curriculum offering over 200 hours of intensive learning, and mastery of 26+ GenAI tools and libraries. Elevate your skills and become a leader in AI. Please rewrite this sentence. Please rewrite the sentence for me.