Introduction
Chain of Questions has revolutionized prompt engineering by introducing a method where each question builds on the previous one, resulting in more profound and insightful responses. This technique enhances AI models’ capability to tackle complex problems by asking interconnected questions, leading to detailed and comprehensive answers. Let’s explore how the Chain of Questions is transforming the landscape of AI systems today.
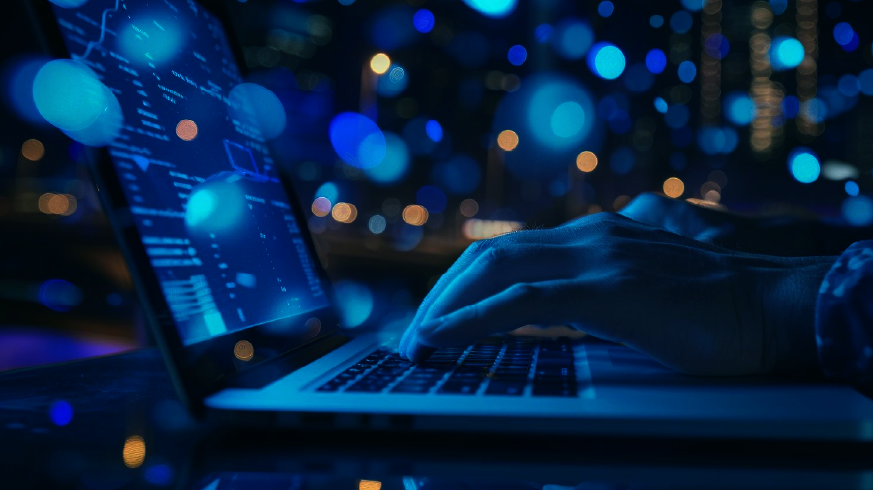
Overview
- Chain of Questions enhances AI prompt engineering by generating interconnected and in-depth responses.
- This technique emulates human inquiry by structuring questions sequentially for detailed analysis.
- CoQ utilizes sequential progression, dependency, and context to guide AI reasoning.
- Implementing CoQ in AI models involves structured prompts and iterative refinement.
- CoQ finds applications in research, journalism, legal discovery, product development, and strategic planning.
- Future advancements in CoQ may include adaptive questioning, multi-perspective analysis, and interactive systems.
Understanding the Chain of Questions
Chain of Questions represents an advanced approach to prompt engineering. In this technique, questions are organized sequentially and interconnected to unravel complex reasoning by AI models. It aims to help AI systems produce intricate and comprehensive results by simulating how humans conduct thorough inquiries or investigations.
The Concept Behind Chain of Questions
The fundamental concept of CoQ revolves around progressive inquiry. In human reasoning, we often start with a broad question and gradually narrow it down to specific aspects based on initial responses. CoQ mirrors this process in AI interactions.
Here’s a breakdown of how it operates:
- Sequential Progression: Questions are logically presented, with each question building upon information gathered from previous responses.
- Dependency and Context: Each question in the chain relies on and contextualizes the next, creating a path for problem-solving.
- Depth and Breadth: The technique allows for both vertical research (digging deeper into specific features) and horizontal expansion (covering various relevant aspects of a topic).
- Guided Reasoning: By breaking down significant themes or issues into smaller, more manageable questions, CoQ guides AI through a systematic reasoning process.
- Iterative Refinement: The chain can include questions that refine or challenge previous responses, leading to a more thorough and accurate analysis.
Implementing Chain of Questions in Prompt Engineering
Let’s utilize the OpenAI API with a well-crafted prompt to demonstrate how we can implement a chain of questions in prompt engineering.
Here’s an example:
Step 1: Install and Import Dependencies
!pip install openai --upgrade
Importing libraries:
import os
from openai import OpenAI
from IPython.display import display, Markdown
client = OpenAI() # Make sure to set your API key properly
Setting Api key configuration
os.environ["OPENAI_API_KEY"]= “Your open-API-Key”
Step 2: Creating Our Helper Function We’ll create a function called generate_responses
The generate_responses function interacts with the API of ChatGPT-3.5 to generate responses.
- It takes a prompt (a question or statement) and the number of desired answers as input.
- It maintains a list to store the responses.
- After obtaining all the answers, it returns a list of responses.
def generate_responses(prompt, n=1):
"""
Generate responses from the OpenAI API.
Args:
- prompt (str): The prompt to be sent to the API.
- n (int): The number of responses to generate. Default is 1.
Returns:
- List[str]: A list of generated responses.
"""
responses = []
for _ in range(n):
response = client.chat.completions.create(
messages=[
{
"role": "user",
"content": prompt,
}
],
model="gpt-3.5-turbo",
)
responses.append(response.choices[0].message.content.strip())
return responses
Step 3: Defining a function (generate_coq_prompt) to create a structured prompt for implementing the Chain of Questions
The generate_coq_prompt function:
- Takes a topic and a list of questions as input.
- Constructs a prompt string using an f-string, incorporating:
- The input topic.
- An instruction to use the Chain of Questions technique.
- The numbered list of questions joined into a single string.
- Instructions for answering each question.
- A request for synthesis and proposing advanced questions.
- Returns the constructed prompt.
def generate_coq_prompt(topic, questions):
prompt = f"""
Topic: {topic}
Using the Chain of Questions technique, provide an in-depth analysis of {topic} by answering the following questions in order:
{' '.join([f"{i+1}. {question}" for i, question in enumerate(questions)])}
For each question:
1. Provide a comprehensive answer.
2. Explain how your answer relates to or builds upon the previous question(s).
3. Identify any new questions or areas of inquiry that arise from your answer.
After answering all questions, synthesize the information to provide a comprehensive understanding of {topic}.
Finally, propose three advanced questions that could further deepen the analysis of {topic}.
"""
return prompt
Step 4: Setting up our topic and questions, creating a prompt, and generating responses
Now, let’s set up our topic and questions, create a prompt, and generate responses using the functions we’ve defined.
- We define our main topic (Artificial Intelligence Ethics) and a list of questions related to this topic.
- We generate a detailed prompt using our generate_coq_prompt function, structuring the Chain of Questions approach.
- We call the generate_responses function with our prompt, interacting with the OpenAI API to obtain solution(s).
- Finally, the code displays the responses. If multiple responses are requested, a loop handles each response, formatting it as a Markdown heading and text for better readability.
topic = "Artificial Intelligence Ethics"
questions = [
"What are the primary ethical concerns surrounding AI development?",
"How do these ethical concerns impact AI implementation in various industries?",
"What current regulations or guidelines exist to address AI ethics?",
"How effective are these regulations in practice?",
"What future challenges do we anticipate in AI ethics?"
]
coq_prompt = generate_coq_prompt(topic, questions)
responses = generate_responses(coq_prompt)
for i, response in enumerate(responses, 1):
display(Markdown(f"### Chain of Questions Analysis {i}:\n{response}"))
Output
The AI systematically addresses each question, providing a comprehensive analysis of artificial intelligence ethics.
The response aligns with the requested structure, addressing each point systematically. It explores the applications of Chain of Questions in various fields like academic research, investigative journalism, legal discovery, product development, and strategic planning. The example provided focuses on environmental policy analysis, demonstrating how the Chain of Questions technique can be implemented. The code snippet shows how to create a structured prompt for policy analysis using a function and generate responses using the OpenAI API.
The output of the analysis showcases a detailed examination of carbon pricing mechanisms, covering different aspects and providing recommendations for future research. The benefits of using Chain of Questions in prompt engineering, such as depth of analysis, structured thinking, uncovering hidden facets, improved problem-solving, and enhanced learning, are highlighted. Additionally, challenges like question selection bias, cognitive load, time constraints, and tunnel vision are discussed.
Looking ahead, the future of Chain of Questions in prompt engineering is envisioned to include adaptive questioning, multi-perspective CoQ, interactive CoQ, cross-domain CoQ, and meta-cognitive CoQ. These advancements will further enhance the capabilities of AI systems in conducting thorough analyses. In conclusion, the Chain of Questions technique proves to be a valuable asset for prompt engineers, enabling them to delve deeper into complex topics and generate insightful analyses. As we enhance these methods, we are not just enhancing AI’s analytical capabilities – we are also laying the foundation for more advanced and context-aware AI interactions that can truly enhance human inquiry and decision-making processes.
Would you like to excel in Prompt Engineering? Enroll in our GenAI Pinnacle Program today!
## Frequently Asked Questions
**Q1. What is a Chain of Questions (CoQ) in prompt engineering?**
Ans. CoQ is a technique that organizes queries sequentially and interconnectedly to guide AI models through complex reasoning processes. It imitates human-like inquiry to elicit more detailed and comprehensive responses.
**Q2. How does a Chain of Questions work?**
Ans. CoQ functions by presenting questions in a logical order, with each question building on previous answers. It involves sequential progression, dependency and context, depth and breadth exploration, guided reasoning, and iterative refinement.
**Q3. What are the main benefits of using a Chain of Questions?**
Ans. The primary advantages include depth of analysis, structured thinking, uncovering hidden facets of a topic, enhanced problem-solving, and improved learning through interconnected questions.
**Q4. What are some applications of a Chain of Questions?**
Ans. CoQ can be utilized in various fields such as academic research, investigative journalism, legal discovery, product development, strategic planning, and environmental policy analysis.
**Q5. What challenges are associated with using a Chain of Questions?**
Ans. Challenges include potential question selection bias, increased cognitive load, time constraints, and the risk of tunnel vision by focusing too narrowly on preset questions. Please rewrite this sentence.