In the field of machine learning, there are various methods for constructing models. One of these methods is ensemble learning, which involves combining multiple models to achieve better performance. By aggregating different predictions, ensemble models can deliver superior results compared to individual models.
This blog post will delve into the workings of ensemble learning, explore different types of ensemble learning algorithms, and discuss the advantages and disadvantages of using ensemble learning for various tasks. This piece is part of our series dedicated to different types of machine learning.
For more context, feel free to explore our other articles:
What Is Supervised Learning?
What Is Unsupervised Learning?
What Is Semi-Supervised Learning?
Reinforcement Learning: How It Works
What is ensemble learning?
Ensemble learning is a machine learning approach that suggests using multiple models to create a more robust model. The fundamental concept behind ensemble learning is that a group of models, when working together, can outperform any single model. It’s akin to teamwork in a corporate setting, where a team of specialists can potentially generate a more qualified solution than an individual employee.
For instance, a common approach in ensemble learning is to utilize decision trees. Instead of relying on the predictions of a single decision tree, the ensemble considers predictions from several decision trees. These trees engage in a voting process where the outcomes with the majority of votes prevail.
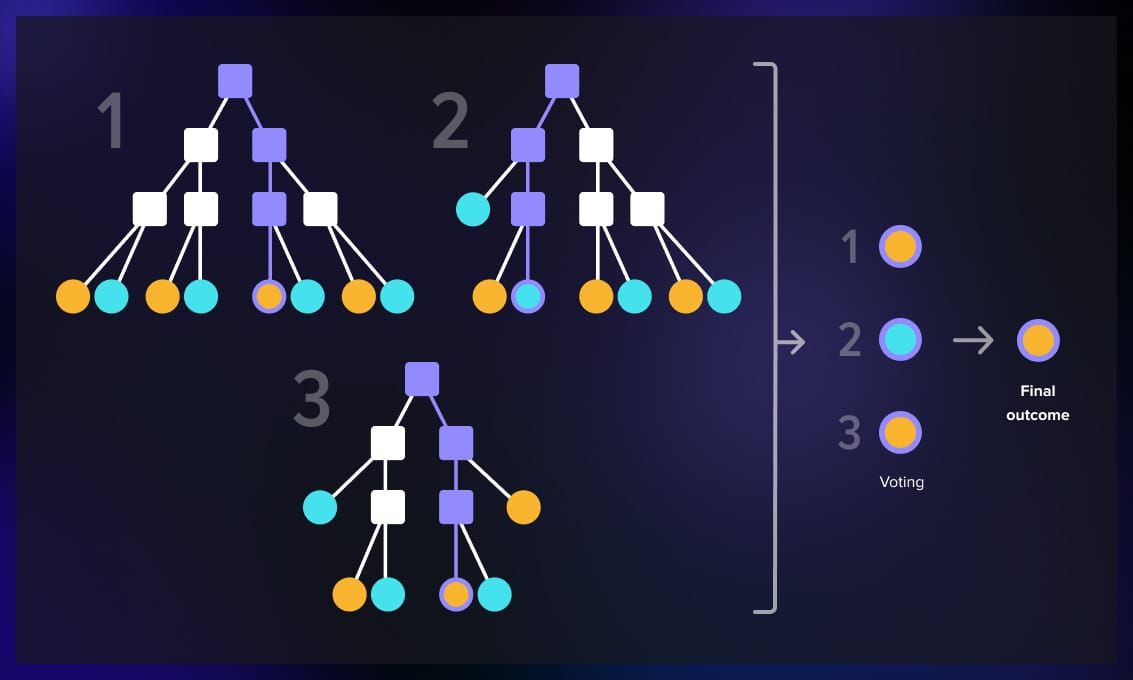
How does it work?
Ensemble learning involves aggregating different machine learning models within a cluster to address a specific problem. This can include several decision trees, neural networks, or a mix of classification and regression algorithms. While these models are trained on the same data, the dataset can also be divided into smaller subsets, or the training can occur sequentially. Ultimately, the outputs of these models are combined and evaluated.
Diversity in models
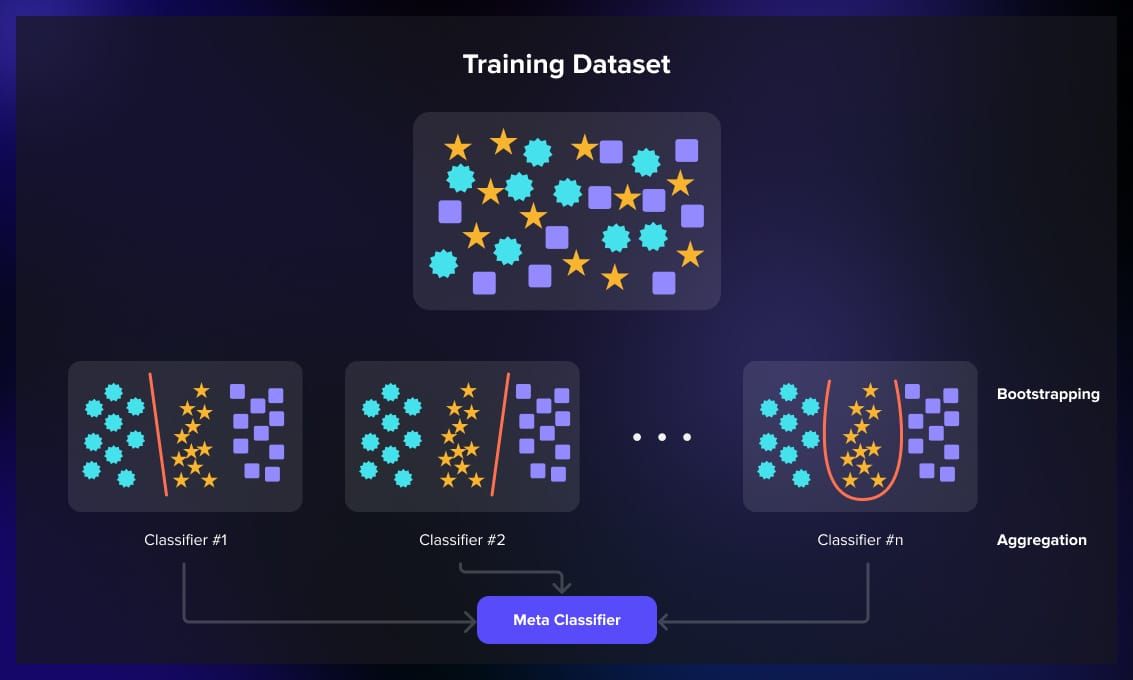
For ensemble learning to be effective, it’s crucial to utilize diverse models. If all models within the ensemble are similar, they are likely to make similar errors. Different models capture various aspects of the underlying data patterns, and their errors do not align. As a result, when combined, the strengths of one model can compensate for the weaknesses of another.
For example, in a classification task with a small dataset, one can employ multiple algorithms such as k-nearest neighbors, SVM, or different decision trees with varying parameters to enhance prediction accuracy.
Soft and hard voting
Ensemble learning models rely on a voting mechanism to determine the final outcome. There are two voting strategies: soft voting and hard voting.
- In hard voting, each model in the ensemble makes a prediction, and the final prediction is based on a majority vote.
- In soft voting, each model in the ensemble provides a probability estimate for each class. The final prediction is then calculated based on the average of these probabilities or values.
Hard voting is typically used for predicting class labels, while soft voting is applied when the base models offer probability estimates or confidence scores.
Ensemble learning techniques
Ensemble learning encompasses various techniques that can be employed for different scenarios.
Bagging (Bootstrap Aggregating)
Bagging entails training multiple instances of the same learning algorithm on different subsets of the training data.
The dataset is segmented into smaller samples, and each model is exposed to a random sample of the data. The predictions are then averaged to produce the final output.
Imagine having a large dataset that is divided into smaller portions and fed into several similar models simultaneously. Each model generates a specific output, which is either voted upon for classification tasks or averaged for regression tasks.
For instance, consider a scenario where you need to separate oranges and apples into different baskets.
Model 1 | Model 2 | Model 3 | Model output | |
Sample 1 | apple | orange | apple | apple |
Sample 2 | orange | orange | orange | orange |
Sample 3 | apple | ? | apple | apple |
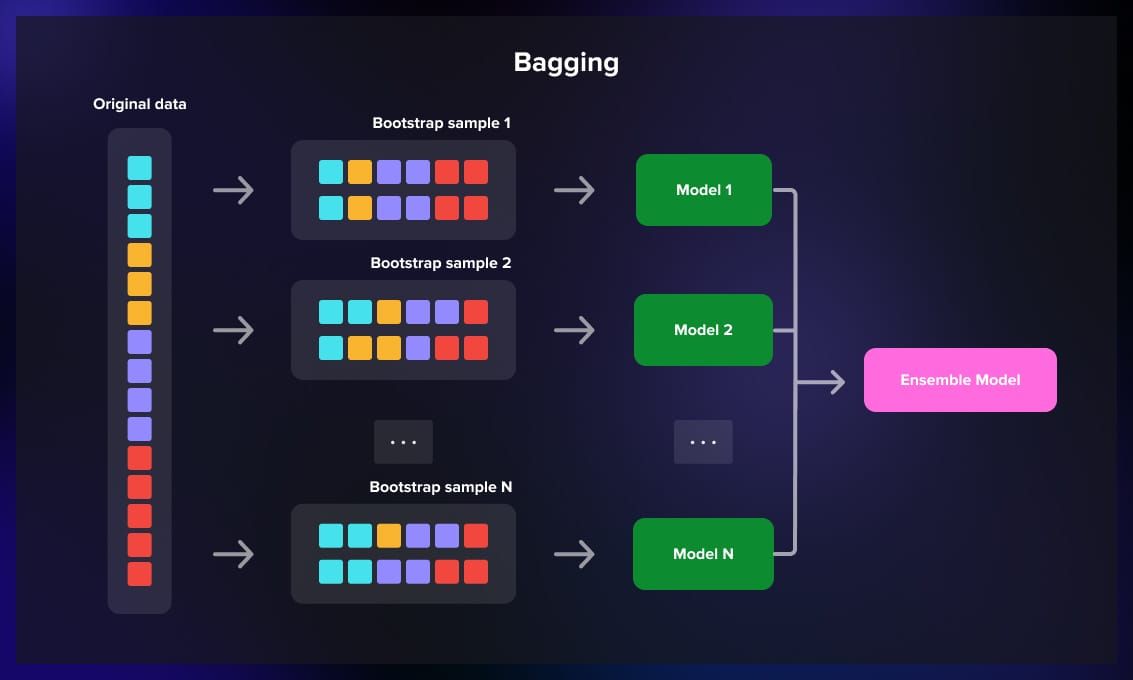
Bagging is beneficial when dealing with a high-variance model and aiming to reduce overfitting.
Example tasks:
- Utilizing decision tree-based models in high-dimensional data, such as random forest for classification or regression.
- Creating an ensemble of neural networks for image classification.
Boosting
Boosting concentrates on enhancing the performance of a weak learner across iterations. Weak learners are models that perform slightly better than random chance.
To boost their performance, machine learning practitioners can leverage algorithms like AdaBoost and Gradient Boosting, where each model is trained sequentially. A subset of training data is fed into the model during training, and the model generates incorrect predictions. The data is then passed to a different weak learner, and this process is reiterated for several iterations. Ultimately, the model produces the overall prediction based on the previous iterations.
The focus here is on rectifying errors made in the earlier steps. The final prediction is a weighted sum of the individual predictions, with more weight assigned to well-performing models.
Visualize the same fruit basket classification task:
Model 1 | Model 2 | Model 3 | Model output | |
Sample 1 | false | true | true | true |
Sample 1 | false | false | true | true |
Sample 1 | false | false | true | true |
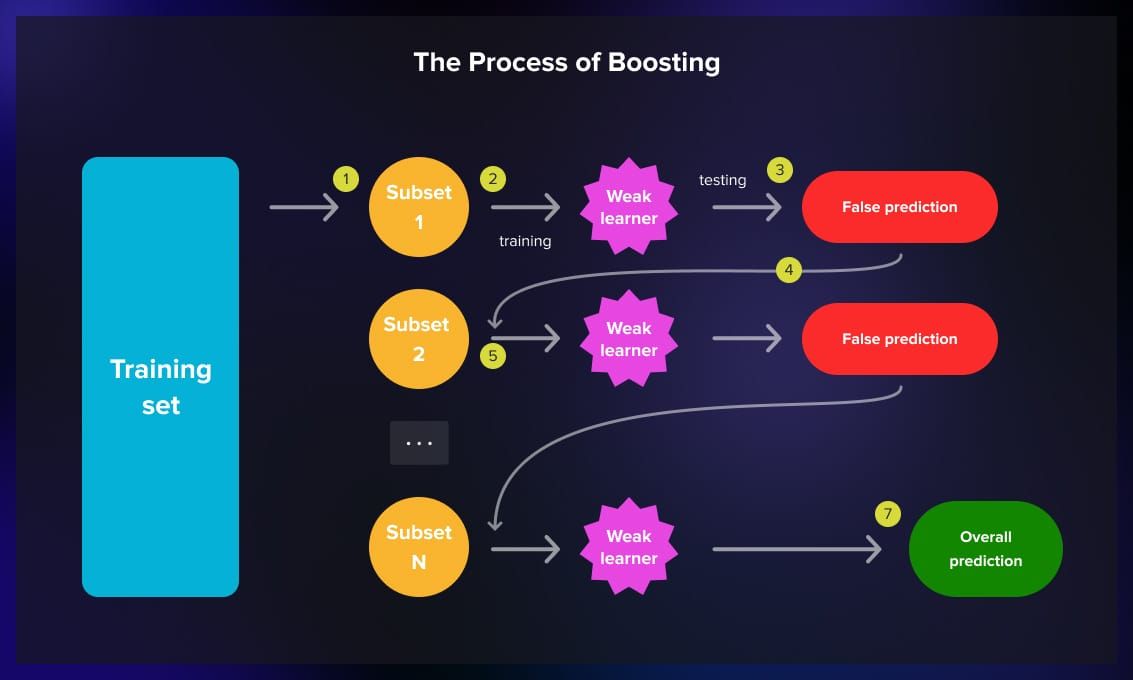
Boosting is employed when dealing with a weak base model and aiming to transform it into a strong model.
Example tasks:
- Addressing classification problems where the objective is to enhance accuracy, such as in fraud detection or spam filtering.
- Tackling regression problems where the goal is to predict a continuous variable, like forecasting house prices.
Stacking
Stacking involves a meta-learner, which is another model that takes the outputs of the base models as input.
This technique is typically used when the models within the ensemble possess different capabilities, and their errors are uncorrelated.
The meta-learner then generates the final prediction based on the combined outputs. Stacking proves effective when diverse base models specialize in capturing various aspects of the underlying data patterns.
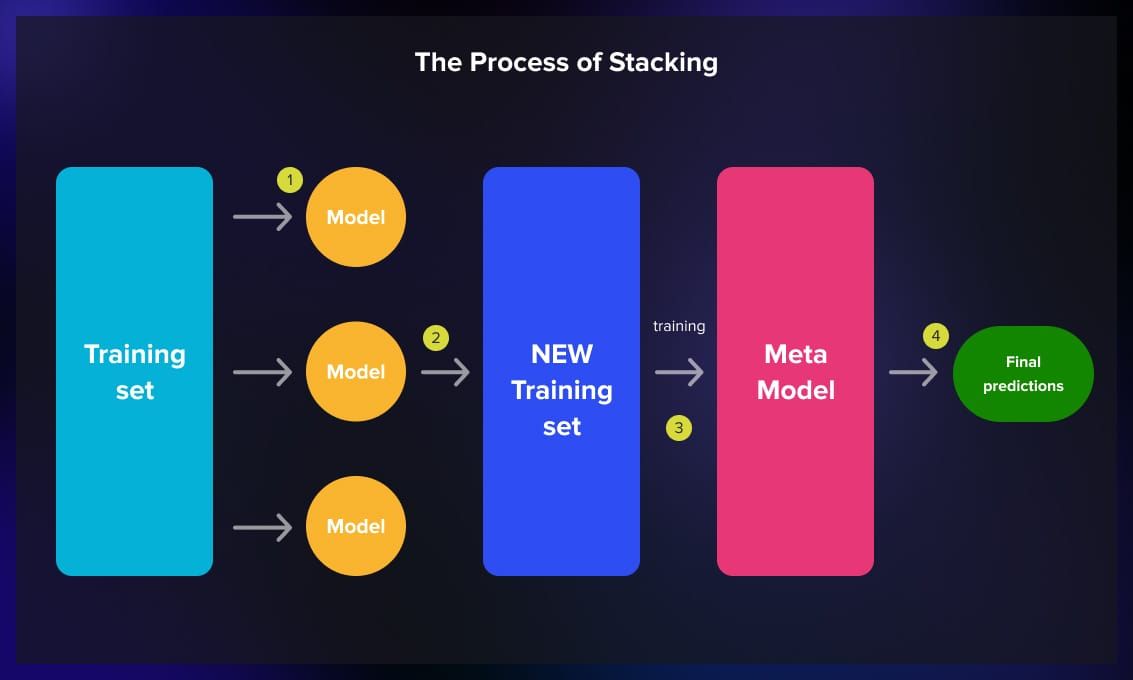
Stacking is implemented when there is a need to merge the predictions of multiple models, leverage the strengths of individual models, and enhance overall performance.
Example tasks:
- Tackling complex problems where different models might excel in distinct areas, such as combining a decision tree model with a neural network to handle a diverse set of features.
- Addressing multi-modal tasks where different models handle various data types (e.g., text, images).
Types of ensemble learning algorithms
Ensemble learning is commonly utilized for classification and regression tasks. For each task, there are numerous algorithms to choose from, based on the problem type, dataset size, and desired trade-offs between interpretability, speed, and accuracy.
1. Random forest
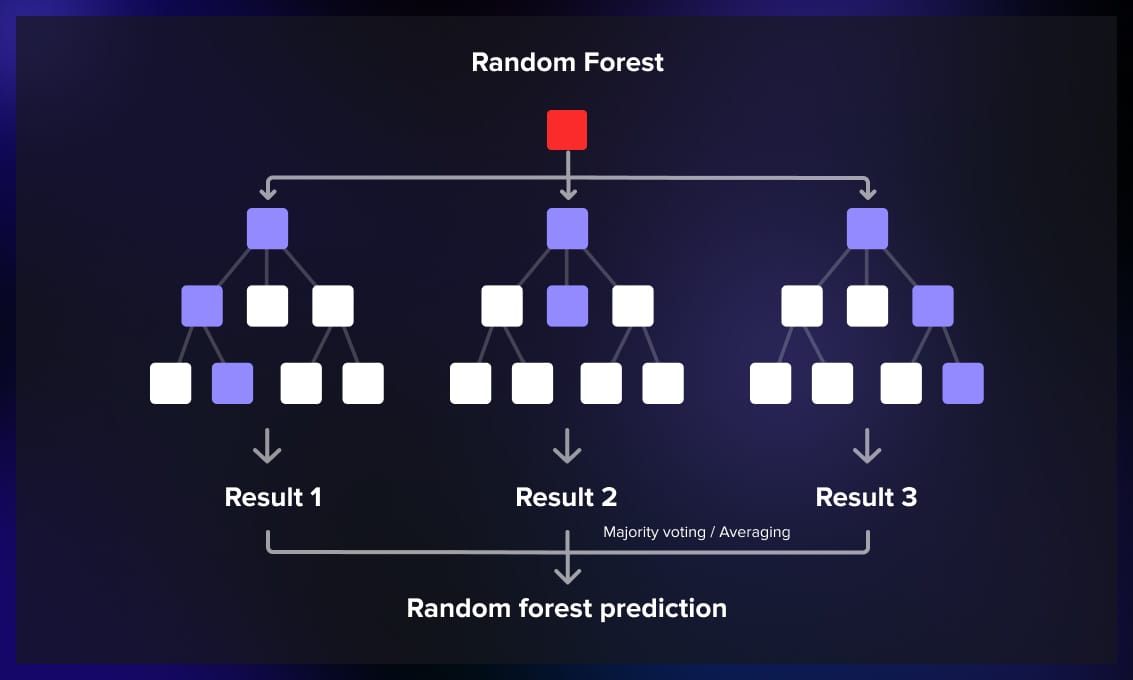
Random forest constructs multiple decision trees during training and combines their predictions. Each tree is trained on a random subset of the training data, and the final prediction is determined by a majority vote. It can be applied to classification, regression, and other tasks.
2. AdaBoost (Adaptive Boosting)
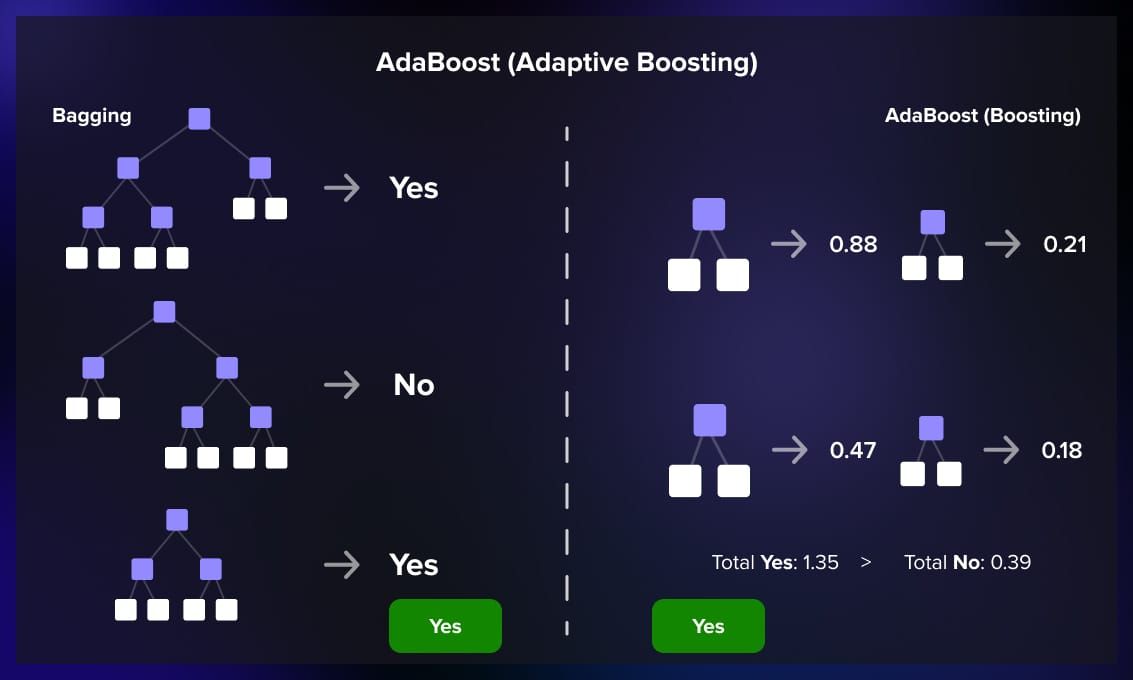
AdaBoost assigns weights to misclassified instances and focuses on training subsequent models to rectify these errors. The final prediction is a weighted sum of the individual model predictions. It is utilized to address the challenge of weak learners in regression problems.
3. Gradient boosting
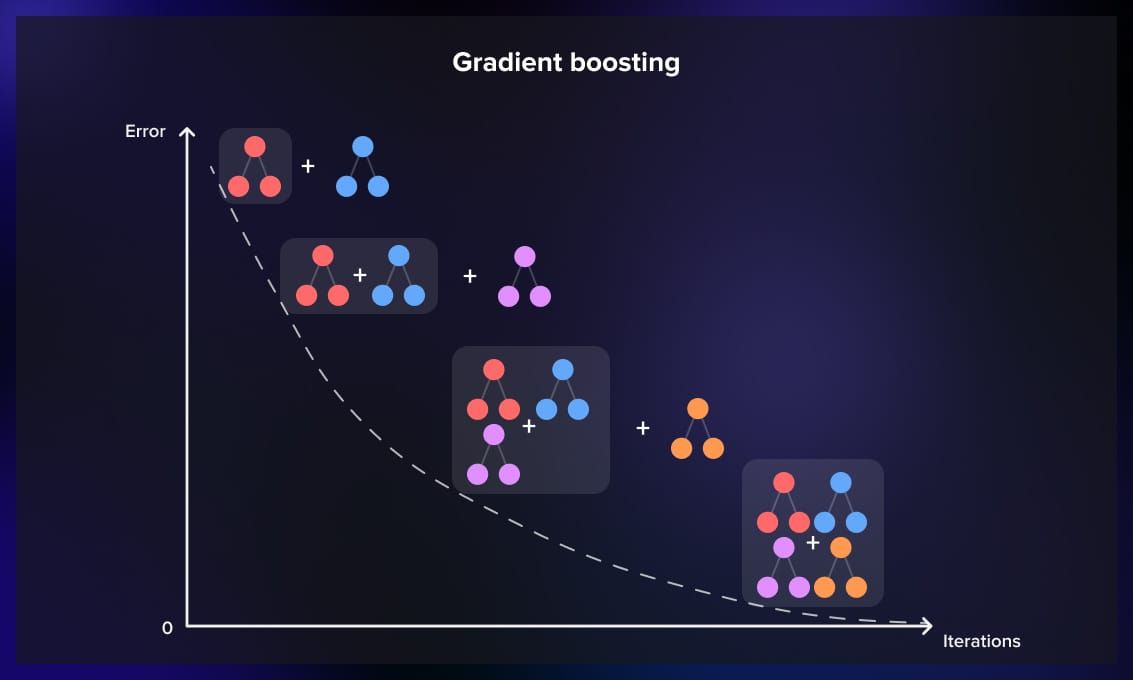
Gradient boosting constructs trees sequentially, with each tree correcting the errors of the previous one. It minimizes a loss function, often leveraging gradient descent, to identify the optimal weights for each tree.
This approach is frequently employed to tackle the issue of weak learners and is suitable for both regression and classification tasks.
4. Bagging Meta-Estimator
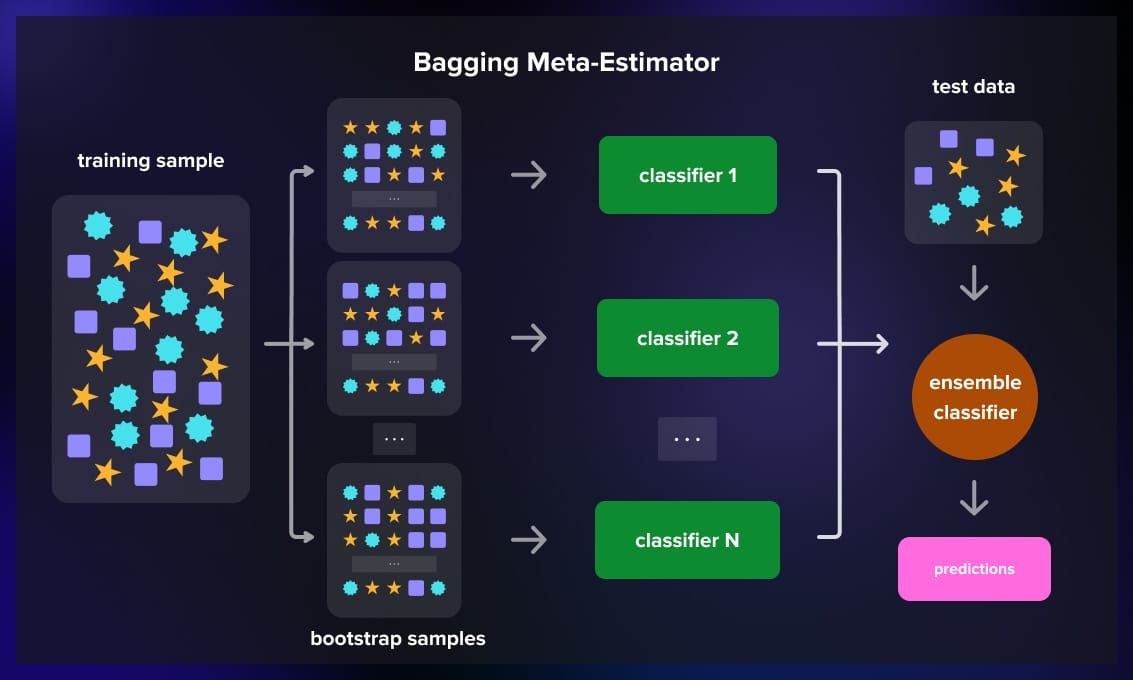
BaggingClassifier and BaggingRegressor provide a general framework for bagging. They can be paired with various base classifiers or regressors.
5. VotingClassifier
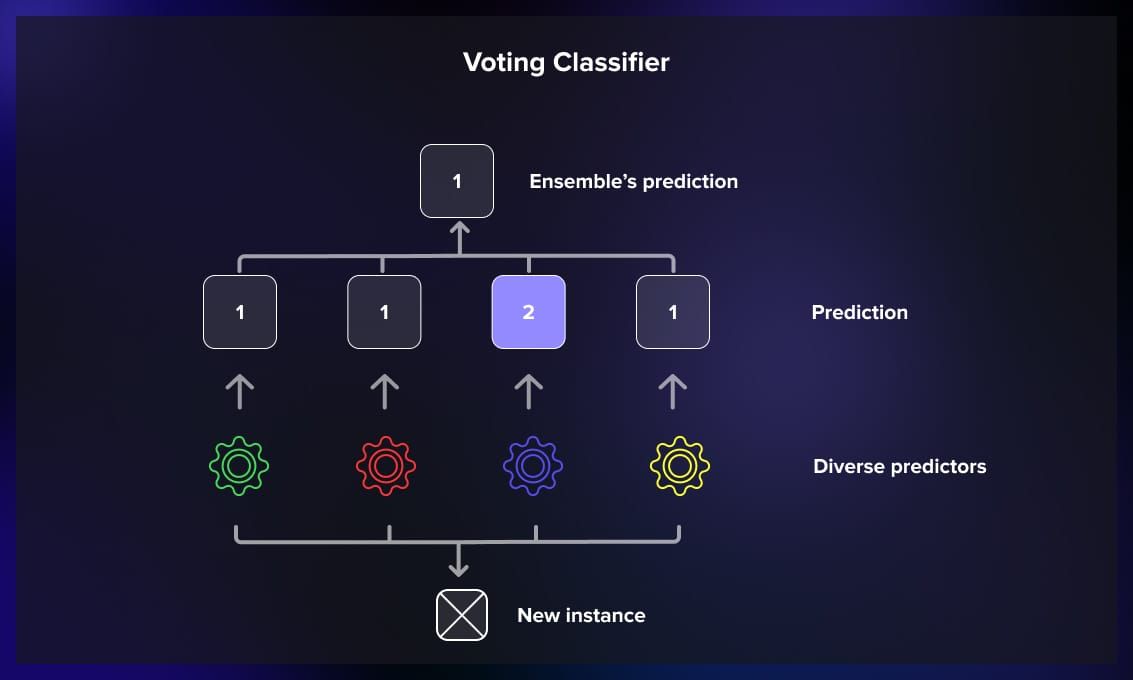
VotingClassifier enables the combination of multiple classifiers (e.g., SVM, random forest, logistic regression) through majority voting. It supports both soft and hard voting strategies.
6. StackingClassifier, StackingRegressor
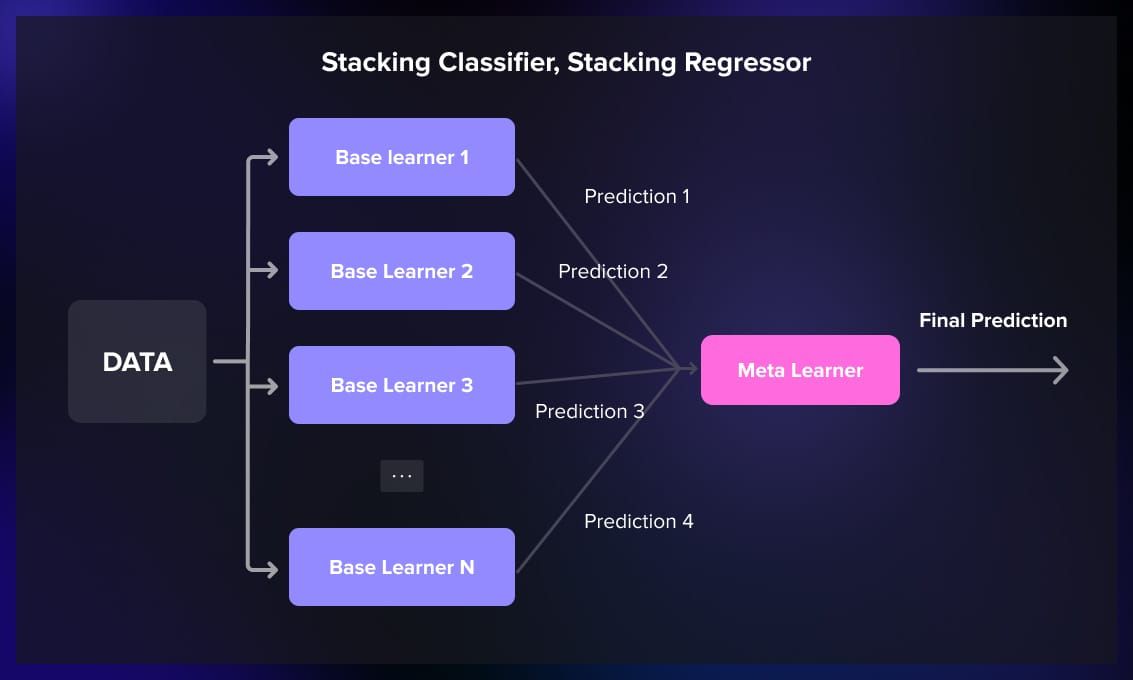
StackingClassifier and StackingRegressor allow for stacking with multiple base learners and a meta-learner. They provide a flexible way to experiment with stacking in a scikit-learn compatible interface.
7. Neural networks
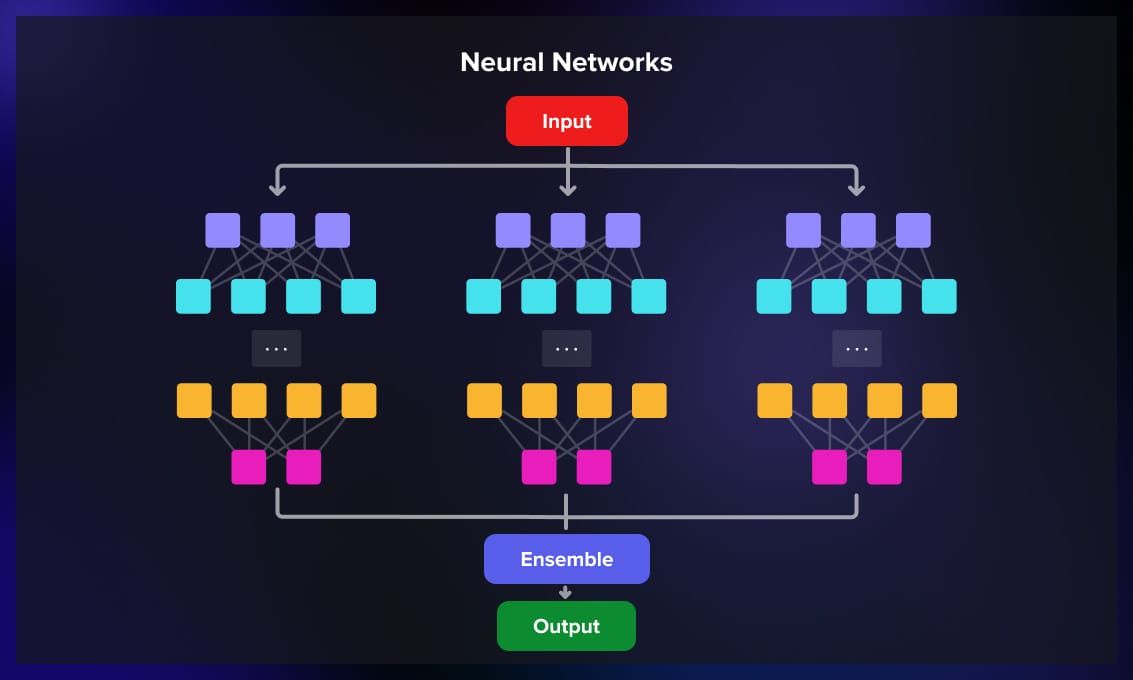
Neural networks can also be integrated into an ensemble. One can employ bagging and train multiple networks independently, then combine their predictions.
Pros and cons of ensemble learning
This section will examine the advantages and disadvantages of ensemble learning models.
Advantages of ensemble learning
Ensemble learning offers several benefits that contribute to its popularity in the machine learning domain.
1. Increased accuracy
Ensemble learning can boost predictive accuracy. By leveraging the strengths of multiple models, ensemble methods often outperform individual models.
2. Improved robustness
Ensemble learning tends to be more resilient to outliers and noisy data. Outliers that significantly impact the performance of one model may have less influence on the overall ensemble prediction. The diversity among models helps mitigate the impact of errors in individual models.
3. Generalization to new data
Ensemble models often generalize well to new and unseen data. The combination of diverse models aids in capturing complex patterns within the data.
4. Versatility across tasks
Ensemble learning techniques can be applied to various machine learning tasks, including classification, regression, and even unsupervised learning. They are adaptable to different types of models and datasets.
Disadvantages of ensemble learning
However, when utilizing ensemble learning, there are also certain drawbacks and trade-offs that practitioners need to consider.
1. Computational complexity
Ensemble methods can be computationally intensive, particularly when dealing with a large number of models or complex base learners. Training and maintaining multiple models necessitate additional resources.
2. Increased model complexity
The combination of multiple models can result in heightened model complexity, making it more challenging to interpret and grasp the inner workings of the ensemble.
3. Overfitting risk
While ensemble methods can mitigate overfitting in certain scenarios, there is a risk of overfitting to the training data, especially if the base models are overly complex or lack diversity.
4. Dependency on base models
The efficacy of ensemble learning hinges on the quality and diversity of the base models. If the base models are weak or similar, the ensemble may not yield substantial improvements.
Conclusion
To conclude, ensemble learning is a potent technique that excels in terms of accuracy, robustness, and generalization. However, it’s important to be mindful of its potential drawbacks, including increased computational complexity, challenges in model interpretability, and the necessity for thoughtful selection and training of base models.
When deciding whether to employ ensemble learning, it’s vital to consider the specific characteristics of the problem at hand and the available resources. As with any machine learning approach, the choice should be guided by the unique requirements of the task.
If you’re eager to delve deeper into machine learning, feel free to explore our recent articles: