In this article, we present insights from Serokell AI experts regarding their research on drug-disease interactions. The focus was on determining whether a drug has a positive, negative, or neutral impact on treating a specific disease.
Serokell collaborated with Neo7Bioscience, a molecular technology company, and Elsevier, an information and analytics firm specializing in medical and biological research. Using data licensed from Elsevier, our experts developed machine learning (ML) models to forecast interactions between small molecules and diseases.
Drug-disease interaction prediction and biological sequence embedding
The project involved analyzing large datasets derived from numerous research papers, condensed into a graph structure. The dataset, though not as extensive as those in fields like natural language processing (NLP) and computer vision, contained various biological entities such as diseases, proteins, and small molecules, along with different types of connections, including clinical trials and regulations.
Two main tasks were undertaken: drug-disease interaction prediction and biological sequence embedding.
- Drug-disease interaction prediction: This task focused on using graph neural networks to predict interactions between drugs and diseases based on information from clinical trials and other sources.
The dataset, organized as a graph, featured nodes representing drugs and diseases connected by edges indicating known interactions. The goal was to predict unobserved connections by enriching the information with additional node types.
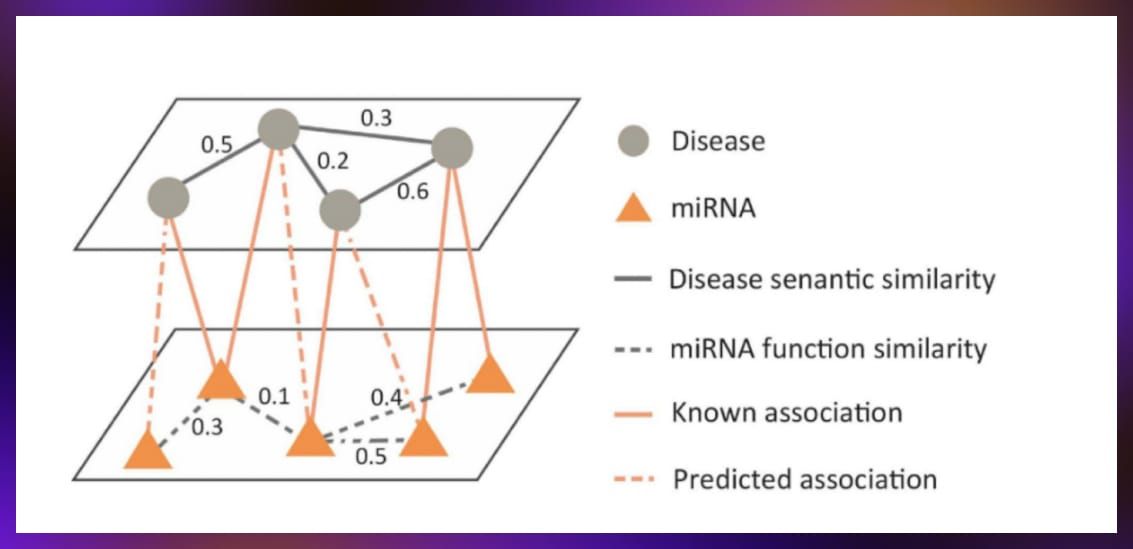
Source
- Biological sequence embedding: This task involved compressing DNA and amino acid sequence information into a vector format for model use. The process included segmenting sequences, generating vectors, and combining them to represent the full sequence’s information, enhancing node information within the graph for improved predictions.
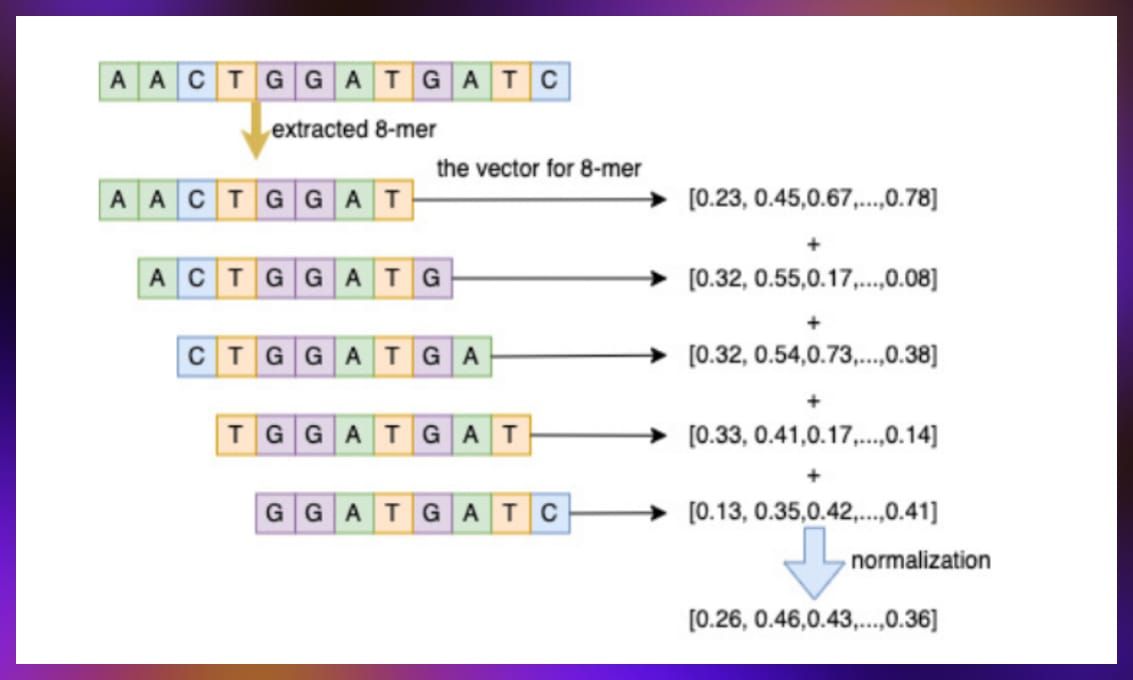
Source
The methodology relied on machine learning graphs, which are further explained in the following sections.
What is graph machine learning?
Graph machine learning processes data in graph formats, leveraging the relationships and structures within graphs to extract insights. This approach combines the power of graphs with machine learning to tackle various tasks such as node classification, link prediction, and graph classification.
Graph neural networks
Graph neural networks (GNNs) have gained popularity in machine learning for their ability to understand complex network structures by incorporating relational information present in graphs. GNNs process graph data composed of nodes, edges, and global attributes, converting them into vector representations for analysis.
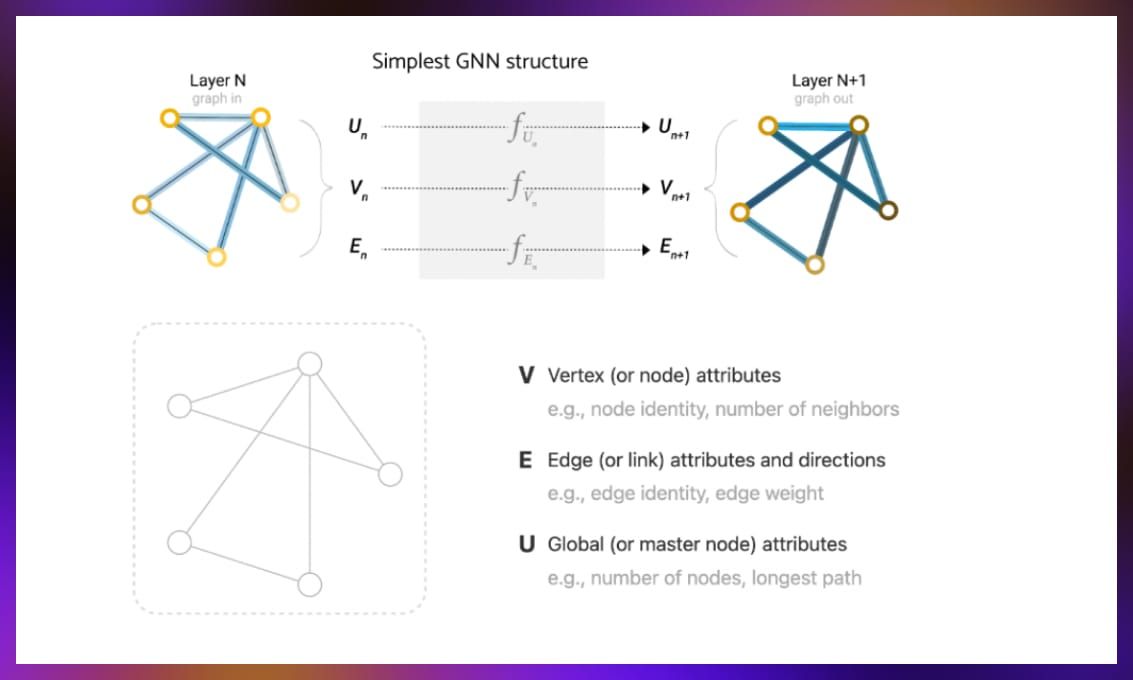
Source
The message passing mechanism in GNNs allows nodes to gather information from neighbors, enhancing the model’s understanding of direct and indirect connections within the graph.
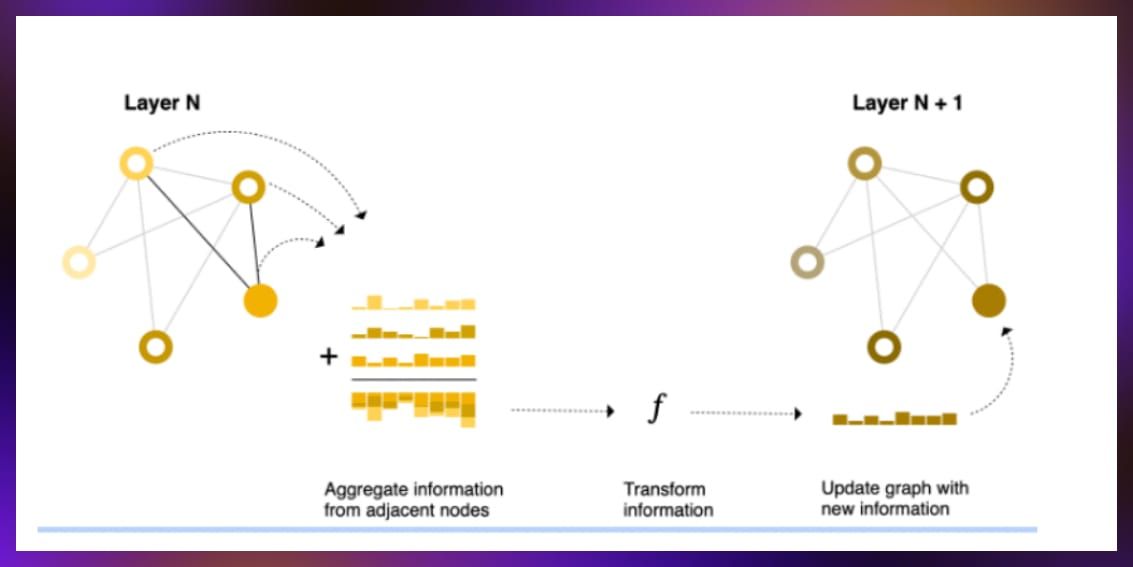
Source
Graph convolutions, a key aspect of GNNs, integrate neighbor information into node representations to learn from complex graph data.
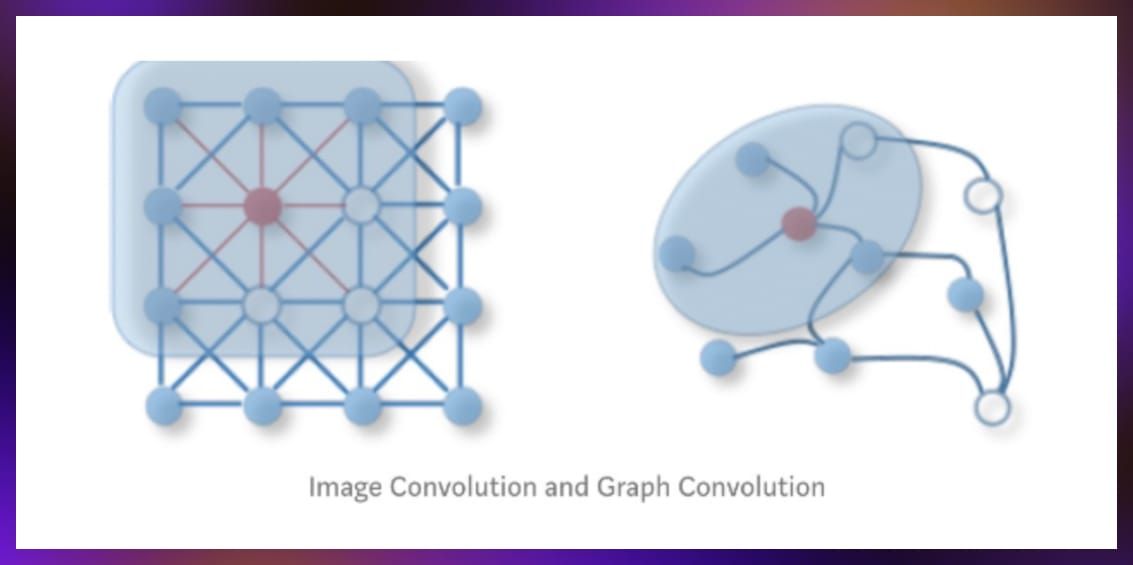
Source
SimpleConv and GraphConv
SimpleConv and GraphConv are operations utilized in graph neural networks to aggregate information from node neighbors for feature updates. SimpleConv is basic and efficient, while GraphConv incorporates trainable parameters for more complex pattern learning.
How are GNNs trained?
Training GNNs involves processing subgraphs instead of the entire graph, with a focus on edge prediction tasks through graph convolutions and node embeddings.
Graph types
The project involved working with heterogeneous and directed graphs, necessitating a distinction between homogeneous and heterogeneous graphs for effective data analysis.
Dense and sparse graph data storage
Two main methods of data storage, dense and sparse, were utilized to represent graph structures efficiently, with sparse storage being preferred for large, sparsely connected graphs.
Directed and undirected graphs
The differentiation between directed and undirected graphs was crucial for modeling relationships and network flows accurately.
Data available in the Elsevier project
The dataset used in the project was heterogeneous and directed, requiring specialized algorithms and models to handle the diverse node and edge types effectively.
Navigating challenges: our progress and future plans
The collaboration faced initial challenges with the dataset and code, prompting a transition to PyTorch Geometric for enhanced graph data management. Future plans involve refining the model with a more comprehensive dataset to evaluate and improve performance.
Stay tuned for updates on our progress in upcoming publications.
Read more:
Drug Repurposing With Graph Neural Networks