Introduction
We are all familiar with Artificial Intelligence, which is reshaping the technological landscape globally and is projected to experience significant growth in the coming years. As AI becomes ingrained in various industries, it is clear that a world without AI will soon be unimaginable. AI is constantly enhancing the intelligence of machines, driving innovations that transform how people work. However, you may wonder, what enables AI to achieve all this and deliver accurate results? The answer is simple: data.
Data serves as the fundamental fuel for AI. The quality, quantity, and diversity of data directly impact the functioning of AI systems. This data-driven approach enables AI to uncover crucial patterns and make decisions with minimal human intervention. However, obtaining large volumes of high-quality real data is often hindered by costs and privacy concerns, among other challenges. This is where synthetic data comes into play.
Learning Objectives
- Understand the significance of synthetic data
- Learn about the role of Generative AI in data creation
- Explore practical applications and their implementation in projects
- Understand the ethical implications of using synthetic data in AI systems
This article was published as a part of the Data Science Blogathon.
The Significance of High-Quality Synthetic Data
Synthetic data is artificially generated data that mimics the statistical properties of real-world data without identifiable distinctions.
Synthetic data is not just a solution for privacy concerns; it is a cornerstone for responsible AI. This type of data generation addresses challenges associated with using real data, especially in cases where data availability is limited or biased. It is also valuable in applications where data privacy is a priority. By mitigating these issues, synthetic data enhances model accuracy.
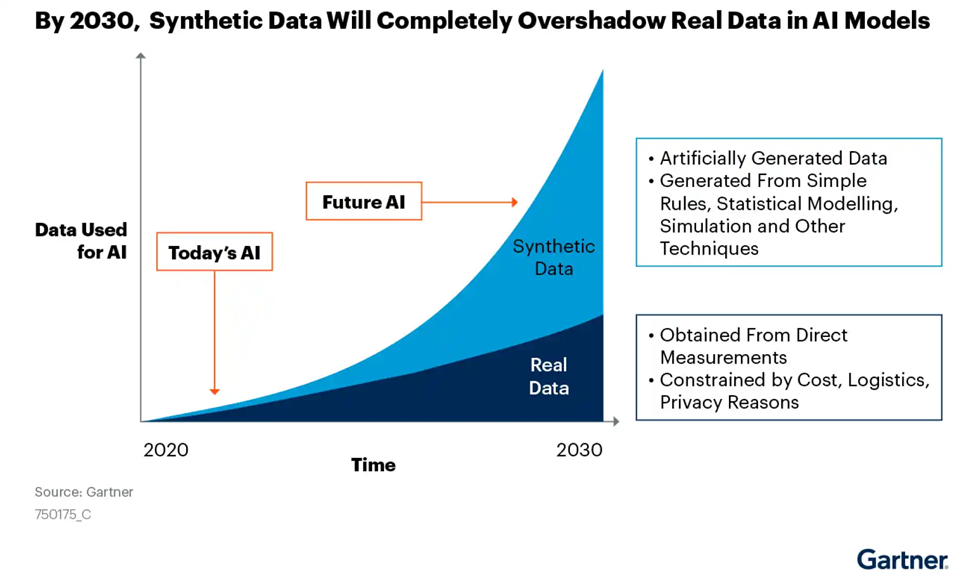
According to a Gartner report, synthetic data is expected to surpass real data in AI model usage by 2030, highlighting its importance in enhancing AI systems.
Role of Generative AI in Synthetic Data Creation
Generative AI models play a crucial role in synthetic data creation by learning patterns from original datasets and replicating them. Through algorithms like Generative Adversarial Networks (GANs) and Variational Autoencoders, Generative AI can generate accurate and diverse datasets essential for training various AI systems.
In the realm of synthetic data generation, innovative tools like YData’s ydata-synthetic and frameworks like DoppelGANger and Twinify stand out for their ability to create high-quality synthetic datasets tailored for specific data science needs. These tools offer solutions for enhancing dataset privacy, expanding data volumes, and improving model accuracy without compromising sensitive information.
Creating High-Quality Synthetic Data
The process of generating high-quality synthetic data involves several key steps to ensure that the data is realistic and maintains the statistical properties of the original data.
It begins with defining clear objectives for the data, such as data privacy, dataset augmentation, or model testing. Analyzing real-world data to understand its patterns, distributions, and correlations is crucial. Various datasets like those from the UCI Machine Learning Repository, Kaggle, and Synthetic Data Vault (SDV) can be utilized to identify statistical properties and generate synthetic data using tools like YData Synthetic, Twinify, and DoppelGANger. Validation of the synthetic data against the original data through statistical tests and visualizations ensures its suitability for applications such as model training, privacy-preserving analysis, and more.
Potential Application Scenarios
Let’s explore potential application scenarios.
Data Augmentation
Data augmentation is a key application scenario for synthetic data, particularly in cases of scarce or imbalanced data. By augmenting existing datasets with synthetic data, AI models can be trained on more extensive and diverse datasets. This application is vital in fields like healthcare, where diverse datasets contribute to robust diagnostic tools.
Below is a code snippet that demonstrates augmenting the Iris dataset with synthetic data generated using YData’s synthesizer, ensuring more balanced data for training AI models.
Synthetic data is important because it allows for the generation of realistic yet private datasets, ensuring compliance with data protection laws and safeguarding sensitive information. It also helps improve the accuracy and reliability of AI models by reflecting the complexity of real-world data, reducing the need for costly data collection and storage, and promoting fairness by creating balanced datasets that mitigate biases. Ultimately, synthetic data plays a crucial role in facilitating responsible, efficient, and ethical AI development. Synthetic data refers to artificially generated data that replicates the statistical characteristics of real-world data but does not contain any identifiable information.
Why is synthetic data important for AI?
Synthetic data plays a crucial role in addressing data privacy, cost, and accessibility issues. It allows AI models to train on extensive and diverse datasets while safeguarding privacy concerns.
How does generative AI create synthetic data?
Generative AI models, such as GANs (Generative Adversarial Networks) and Variational Autoencoders, analyze patterns from real data and reproduce these patterns to generate synthetic data.
What are the practical applications of synthetic data?
Synthetic data can improve the quality and fairness of AI models by expanding data, reducing bias, and upholding privacy when sharing data.
*The media displayed in this article is not owned by Analytics Vidhya and is used at the Author’s discretion.*