Object detection plays a crucial role in computer vision by identifying and locating objects in images through the use of bounding boxes. This task is essential for various applications across different fields such as autonomous driving, drones, disease detection, and automated security surveillance.
Today, we will delve into FCOS, an innovative object detection model that has gained popularity in various domains. Before we explore the advancements of FCOS, it is important to understand the different types of object detection models available.
Types of Object Detection Models
Object detection models can be categorized into two main types: one-stage detectors and two-stage detectors.
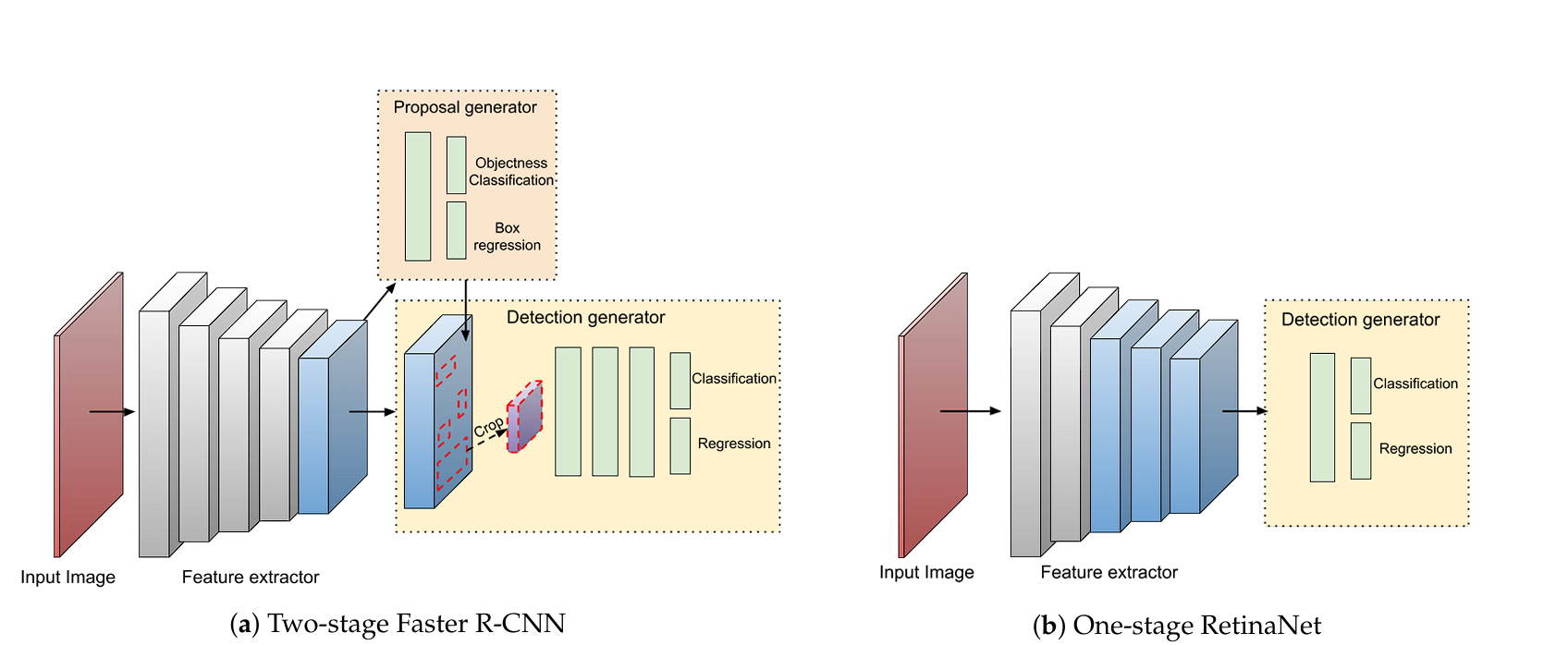
Two-Stage Detectors
Two-stage detectors, such as R-CNN, Fast R-CNN, and Faster R-CNN, break down the object detection process into two stages:
- Region Proposal: The model generates a set of region proposals in the first stage, which are likely to contain objects. This is achieved using methods like selective search (R-CNN) or a Region Proposal Network (RPN) (Faster R-CNN).
- Classification and Refinement: In the second stage, the proposals are classified into object categories and refined to enhance the accuracy of the bounding boxes.
While two-stage detectors are more robust and achieve higher accuracy, they are slower, more complex, and challenging to implement compared to one-stage detectors.
One-Stage Detectors
One-stage detectors, including FCOS, YOLO (You Only Look Once), and SSD (Single Shot Multi-Box Detector), eliminate the need for region proposals. These models directly predict class probabilities and bounding box coordinates in a single pass through the input image.
One-stage detectors are simpler, easier to implement, and significantly faster, making them suitable for real-time applications. Although they may be less accurate and rely on pre-defined anchors for detection, FCOS has narrowed the accuracy gap compared to two-stage detectors and completely eliminated the use of anchors.
Understanding FCOS
FCOS (Fully Convolutional One-Stage Object Detection) is a groundbreaking object detection model that removes the reliance on predefined anchor box methods. Instead, it predicts object locations and sizes in an image directly using a fully convolutional network.
This anchor-free approach in FCOS has led to a reduction in computational complexity and an improvement in performance. Additionally, FCOS surpasses its anchor-based counterparts in terms of performance.
Anchor-Free Detection Explained
In single-stage object detection models, anchors are pre-defined bounding boxes used during both training and inference to predict object locations and sizes in images.
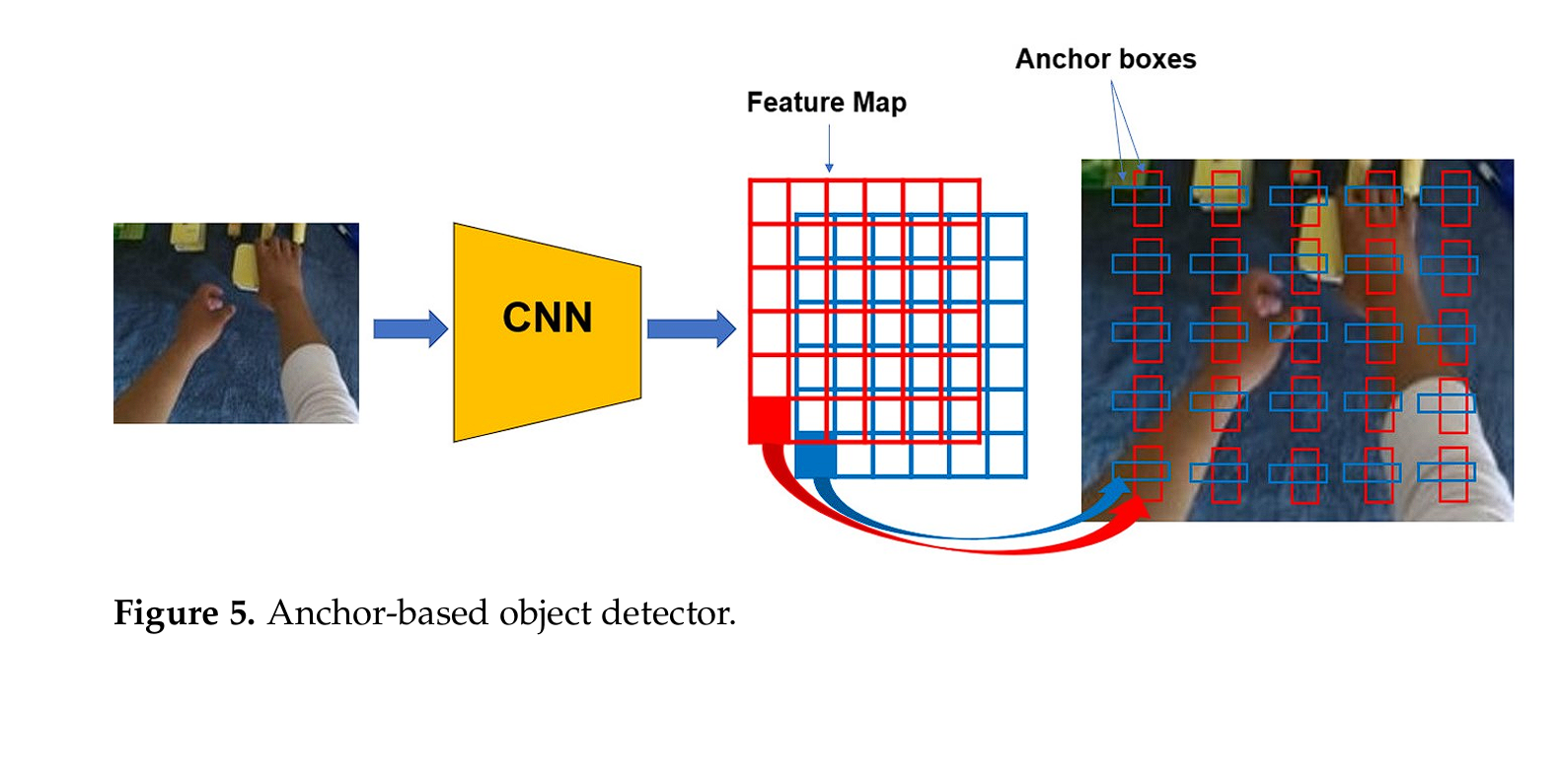
However, anchor-based detectors face challenges such as complexity, computation related to anchors, challenges in anchor design, and imbalance issues. These limitations can impact the performance and efficiency of the model.
FCOS Architecture
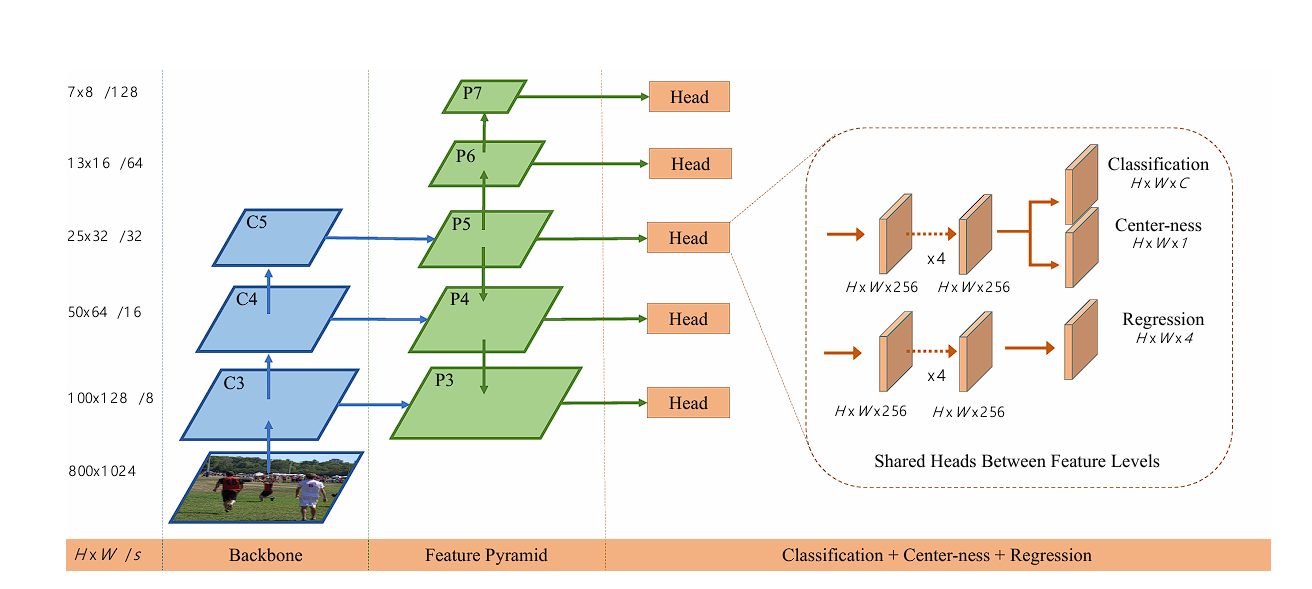
Backbone Network
The backbone network serves as the feature extractor, transforming images into rich feature maps used for detection in the FCOS architecture. In the original FCOS research paper, ResNet and ResNeXt were utilized as the backbone networks.
The backbone network processes input images through multiple convolutional layers, pooling, and activations to extract hierarchical features. These features range from simple edges and textures in early layers to complex object parts and semantic concepts in deeper layers.
The features extracted by the backbone network are then fed into subsequent layers for object detection. This ensures that the prediction features are both spatially accurate and semantically rich, enhancing the accuracy of the detector.
ResNet (Residual Networks)
ResNet incorporates residual connections or shortcuts that skip one or more layers, addressing the vanishing gradient problem and enabling the creation of deeper models like ResNet-50, ResNet-101, and ResNet-152.
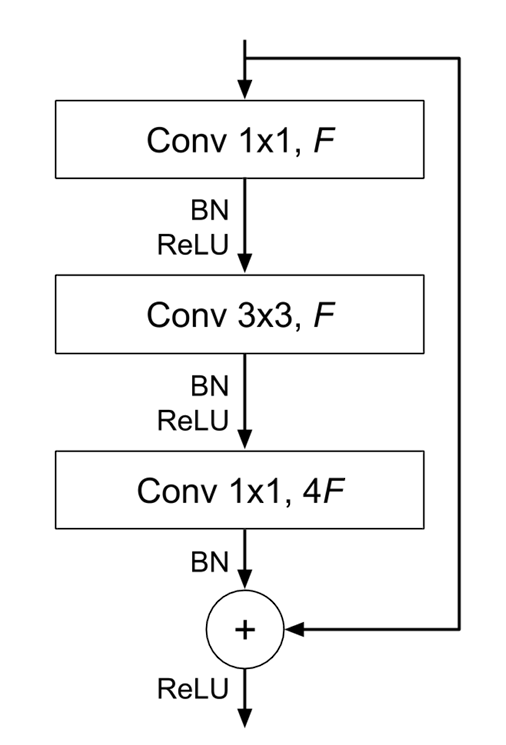
By enabling gradients to flow directly through the network during backpropagation, residual connections help address the challenges associated with training very deep neural networks. In the FCOS research paper, a Feature Pyramid Network (FPN) was also utilized.
What is FPN?
Feature Pyramid Network (FPN) enhances the ability of convolutional neural networks to detect objects at multiple scales. By creating outlets at both initial and deeper layers, FPN enables the detection of objects of various sizes and scales.
Combining features from different levels allows the network to better understand context and separate objects from background clutter. Additionally, FPN’s high-resolution feature maps from early layers enable the detection of small objects, which are challenging to detect in lower-resolution feature maps from deeper layers.
Multi-Level Prediction Heads
In FCOS, the prediction heads are responsible for making object detection predictions. There are three separate heads in FCOS, each performing different tasks on the feature maps generated by the backbone network:
Classification Head
The classification head predicts object class probabilities at each location on the feature map, indicating the likelihood of an object belonging to a specific class.
Regression Head
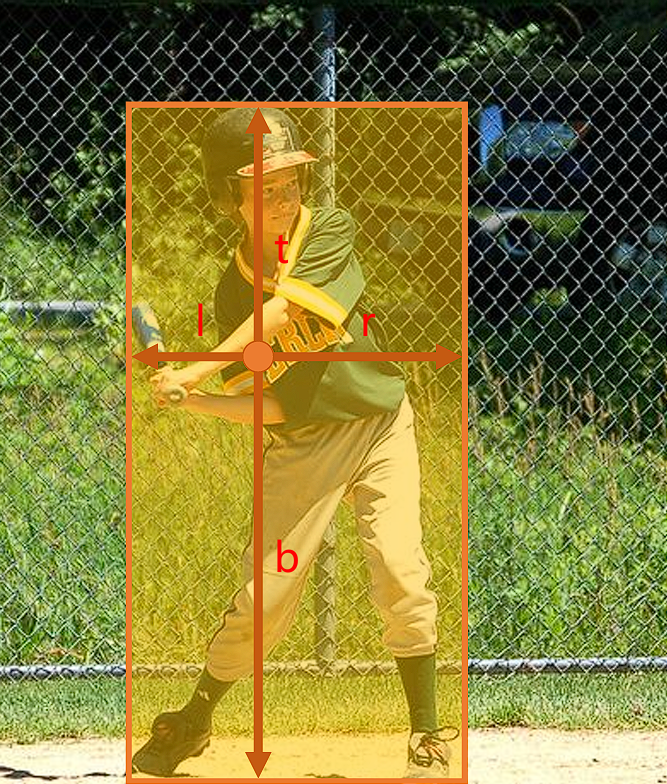
The regression head predicts bounding box coordinates for detected objects at each location on the feature map. It outputs values for the left, right, top, and bottom coordinates of the bounding box, enabling precise object localization without the need for anchor boxes.
For each point on the feature map, FCOS predicts four distance values:
- l: Distance to the left boundary of the object.
- t: Distance to the top boundary of the object.
- r: Distance to the right boundary of the object.
- b: Distance to the bottom boundary of the object.
The predicted bounding box coordinates can be derived using these distances, enhancing the accuracy of object detection.
Center-ness Head
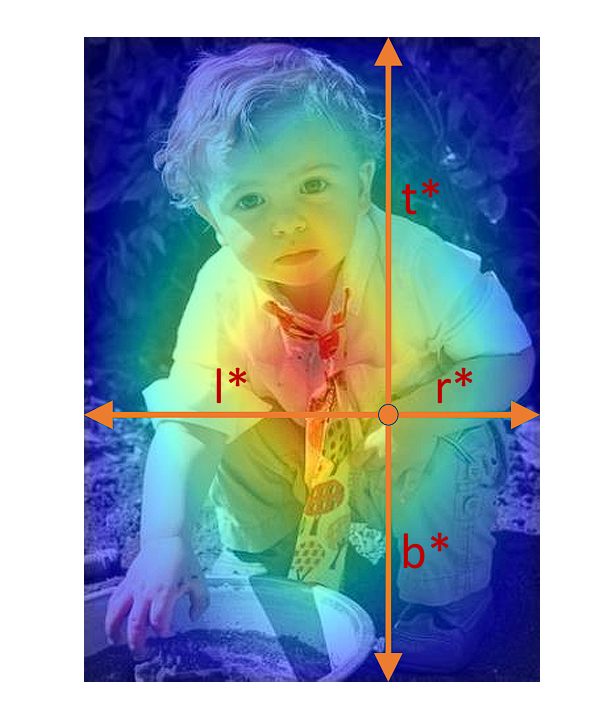
The center-ness head predicts a score indicating the likelihood that a location is at the center of a detected object. This score helps adjust bounding box predictions based on the object’s center, improving the accuracy of object localization.
These prediction heads work together to perform object detection effectively:
- Classification Head: Predicts object class probabilities at each location.
- Regression Head: Provides precise bounding box coordinates for object localization.
- Center-ness Head: Enhances the bounding box predictions by adjusting based on the object’s center.
During training, the outputs from these heads are combined to refine bounding box predictions and eliminate low-quality detections.
The Loss Function
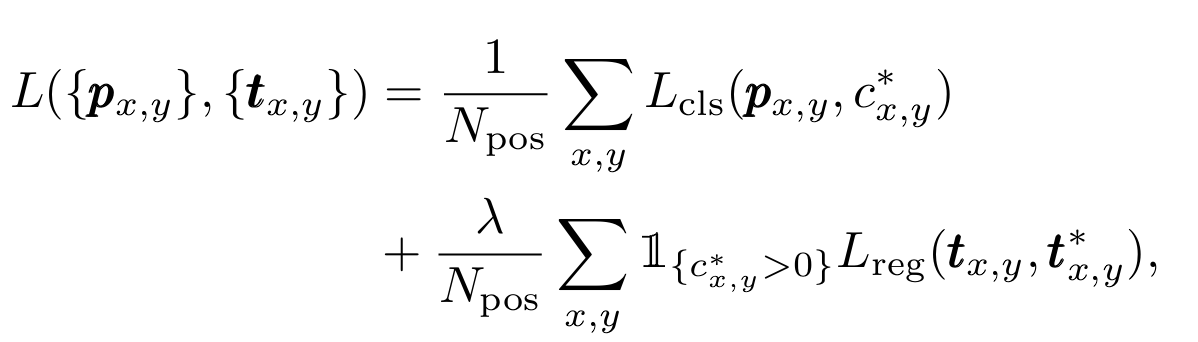
The loss function in FCOS consists of focal loss for classification and regression loss terms. The total loss is calculated by summing these terms to train the model effectively.
Conclusion
Today, we delved into FCOS, a revolutionary object detection model that eliminates the need for predefined anchors, unlike traditional models like YOLO and SSD. By adopting an anchor-free approach, FCOS simplifies object detection processes and enhances computational efficiency.
The FCOS architecture, with its ResNet backbone and multi-level prediction heads, enables accurate object detection by predicting bounding boxes directly without relying on anchors. FCOS sets a strong foundation for future research in improving object detection models.
For more insights into computer vision tasks, explore our other blogs to enhance your knowledge in this field.